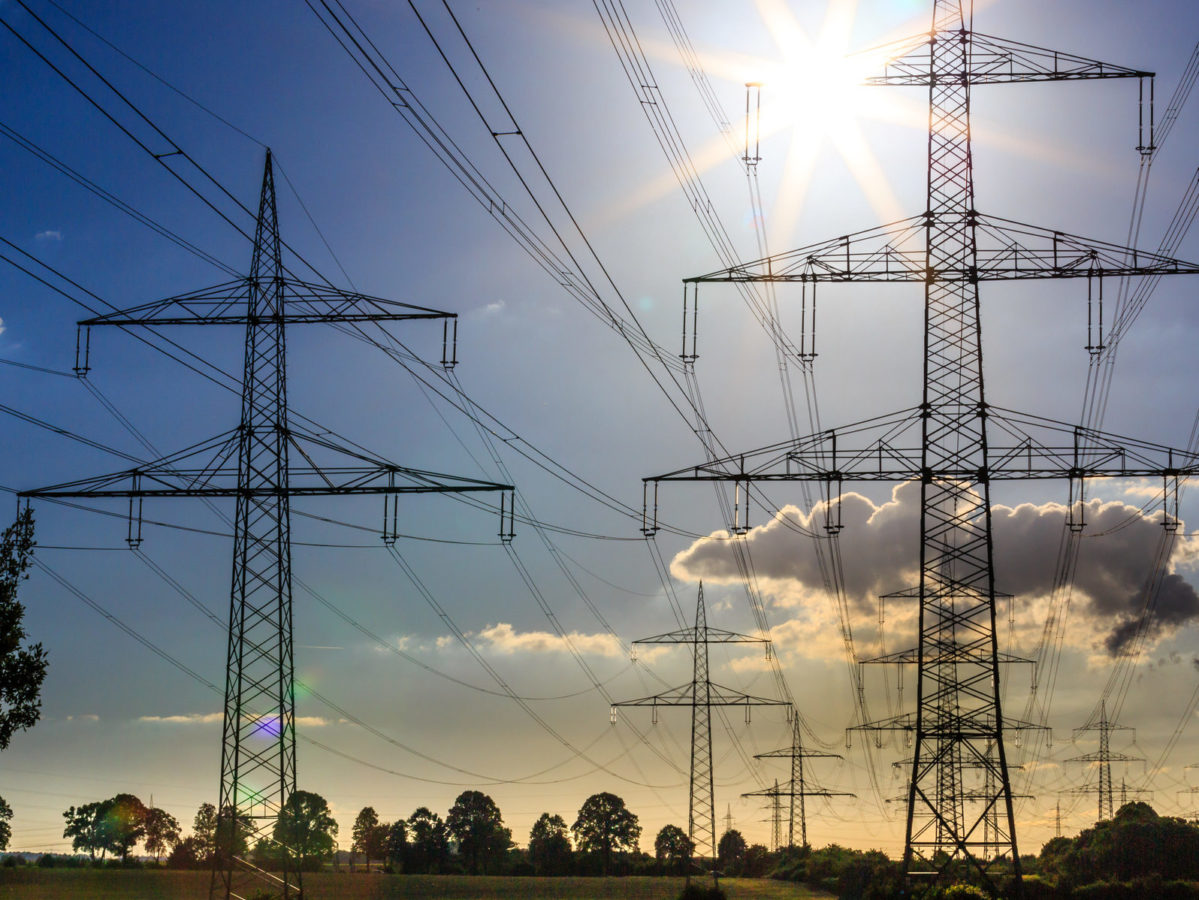
There is a new mindset coming to the forefront for utility leadership, a mindset that says: “Data must be considered a vital asset to the enterprise.” Without this statement, grid modernization efforts will only go so far.
Distribution system operations are increasingly dependent on big data platforms. While utilities are very good at managing physical assets, managing big data analytics involves a relatively new set of processes and procedures. Advanced analytics infrastructure is a key area of importance, along with efforts to optimize the IT/OT infrastructure and organizational processes that support analytics capabilities.
Data Then, and Data Now
As more field devices are added to utility T&D systems, the associated amount of data is increasing exponentially. Utility industry professionals involved in advanced analytics professionals are applying big data and data science methodologies to solve demanding business problems. To do so they must extract meaningful statistical results, analyzing large and complex data sets by performing data quality monitoring and data analysis to database upgrades and facilitate independent and collaborative environments, and by working in cross-functional teams.
Across these and related enterprise analytics areas, a key to exceptional performance will be first and foremost to have a strategy for a data management framework. Such a strategy needs to be informed by advanced analytics considerations, along with systems to manage security, risk, audit, and data profiling functions.
This data framework should optimize how each utility will utilize advanced analytics in the future—notably including the following data-related perspectives of importance:
Allowing for decentralized data vs. focusing on a central data repository
There is a shift underway which involves virtualization of data. It is helpful to think about virtualization as a way to mitigate a lot of movement of data. A lot of data certainly gets moved around in organizations and that is fine, but if data can be leveraged where it is of most value locally, it is of great potential benefit to business users.
Having data go from “mere information” to analytics-driven actionable insights
Large population, relatively inexpensive utility assets, such as pole-mounted distribution transformers, which previously would have been too expensive to monitor individually in real time, are now coming under the domain of IT / OT convergence and big data, so that it will make less and less economic sense to run them to failure. In much the same way that this old utility “run to failure” attitude is becoming a thing of the past; these attitudes about utility data are also on their way out.
Utilities’ time-tested methods for ensuring O&M excellence and high safety and reliability of service evolved over a long period of time. In the industry’s movement toward greater utilization of data-driven solutions, there have been many successful pilots and roll-outs of new technologies to optimize grid operations. But there is a difference between successful pilot programs for new technologies and having robust capabilities that meet utility’s high standards for O&M excellence while fully deploying those new technologies.
This is where advanced analytics and data science are helping optimize the framework for the next wave of processes to support utilities’ O&M excellence. The framework helps utilities in developing capabilities for these new processes and procedures in order to achieve the insights needed from enterprise analytics.
Data governance needs to go beyond an IT focus
A lot of earlier data governance work rightfully had an IT focus. But now utilities need to widen their view by taking a perspective that goes beyond IT. Data must be looked at as it flows between different parts of the distribution network. While most or all data may be seen as filtering through IT, ultimately it is all OT.
Enterprise Data Governance and Data Management Framework Model
As you collect more metadata, whether from SCADA, AMI, ADMS, OMS, or other T&D solutions, the value to the business is increased. But due to the diverse range of systems and the number of different devices providing this data, at times the data is inconsistent. The value of the associated metadata to a utility’s business increases as more data is collected from these devices.
A good enterprise analytics approach will look at what happens when you combine data from these systems with other data to gain deeper insights. The types of data that can be combined is enormous, even just considering existing technologies, let alone emerging technologies.
What are the positive and negative impacts when you start to aggregate such data with the data associated with other assets and systems, and move that data around? What happens when there is a proliferation of underlying IT / OT converged solutions, integrations, communications protocols, etc., to maintain, which multiply every time one such system “talks” with another?
It is important to look at how different technologies interact. The result is that you need to design your framework around how best to utilize data proactively across different devices in the field.
In the increasingly IT / OT converged grid toward which leading utilities are evolving, O&M activities have to include the optimal maintenance of all assets, including the IT assets and related communications and control systems—while this was done in the past with these systems, the benefits of the numerous new devices being installed are going to be increased dramatically by systematically planning future activities associated with optimizing operations and maintenance of those devices.
An important additional element is to ensure good this type of lifecycle management information is integrated into Enterprise Asset Management / Work Management solutions unique to each utility, to inform field work, inventory, and supply chain functions and related standards in order to ensure the infrastructure supporting the new advanced analytics framework is as robust as the overall grid itself, thereby informing related field workflows in this increasingly interconnected set of solutions. OT system data ends up flowing down to IT systems.
Simplify, Simplify
When it comes to optimally managing IT and OT utility assets across the enterprise, utilities have a lot of separate areas that above all require simplification in order to be effective.
In a well-planned and organic way, design efforts should inform and simplify the systems and organizations to which they are applied, minimizing unnecessary complexity by maximizing utilization of standards to drive common specifications, procedures, repositories, data structures, and data dependencies.
As the complexity of these processes increases, we increasingly utilize advanced analytics to simplify how we address them. Along the way, we improve capabilities for statistical interpretation of large volumes of data, and better utilize machine learning techniques to achieve insights from enterprise analytics. But the key to these enterprise analytics improvements is to manage data better and put data management frameworks in place. The outcome will be Data Governance, Quality, Architecture, Operations, Metadata, Master Data, and Privacy driving exceptional Enterprise Analytics, whether using Big Data platforms or general-purpose Data Warehouses.
Security considerations and data privacy concerns add additional layers. They also require that you know where data is, where related supporting assets are, and who has access to them, and who did or did not exercise those access privileges. If the assets to which we are applying security considerations to have already been through an organic design process, then the work is off to a better start. Personnel privileges and training histories and other aspects of security profiles need to be enabled systematically across the enterprise as part of the process of implementing security best practices.