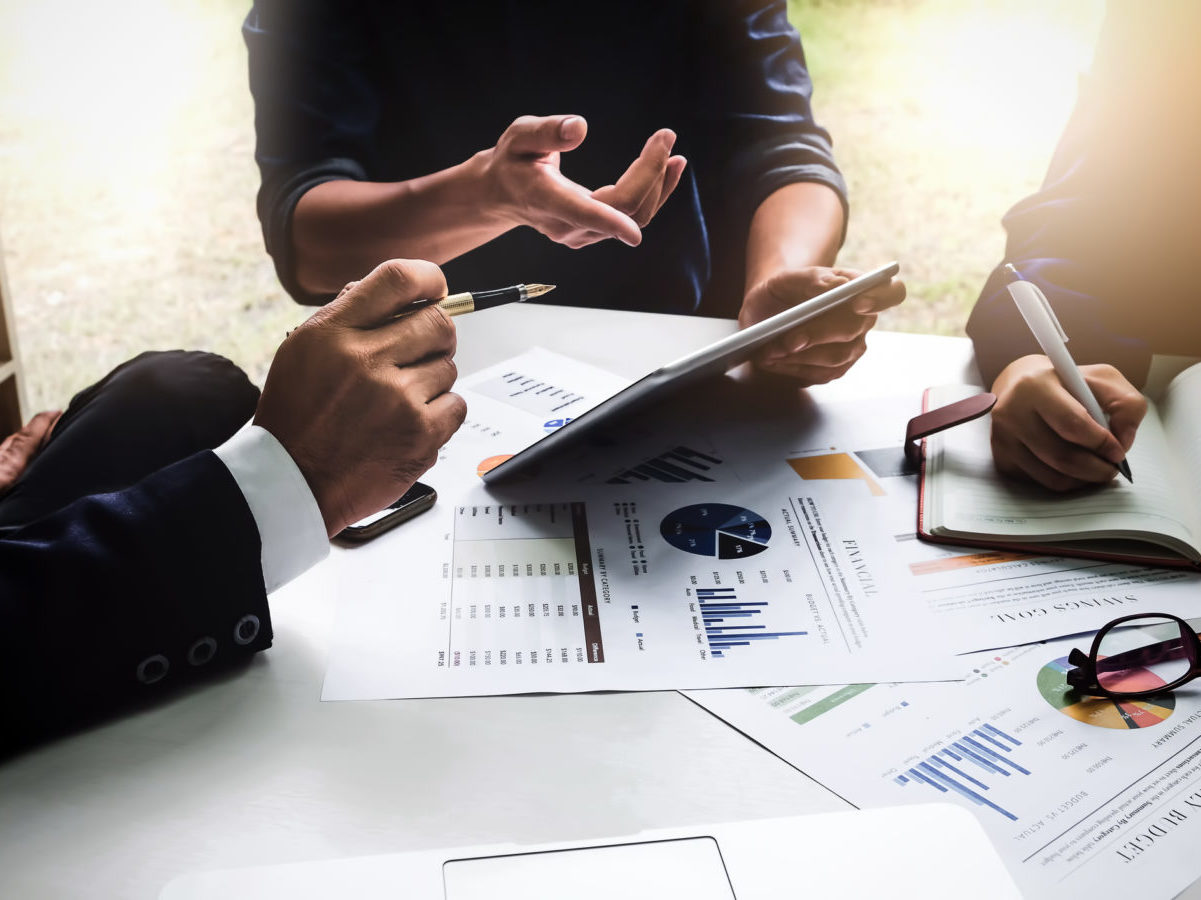
The Analytics Architecture & Technology Advancement Group at UAI meets to share insights and experience on the use of customer segmentation. This is a report out of the discussion.
In an ideal working environment, we have no barriers. We can access information and tools readily that allow us to not only do our job, but also to find ways in which to do so more effectively. That is what analytics is great for. Unfortunately, as many of our members are finding, it’s much easier said than done.
Advanced analytics at most utilities is the grown-up version of siloed excel spreadsheet models or access databases. The difference being that technologies, culture, and process have been optimized to enable users across the utility to efficiently access data, perform analysis, and report findings to others in the company. In an ideal world, this is the analytics self-service holy grail.
In the real world, elements of technology, process, and culture have all improved analytics self-service, but challenges and imperfections in each area, in addition to changing protocols around the use of data, pose limitations. As a result, many users find it more efficient to remain siloed, and maintain dependence on imperfect tools.
Upon the evolution of modern data environments and data lakes, a lot of issues around data governance occur, things like ownership, quality, and accessibility of data. These are all major barriers to self-service across many utilities.
As well, many utilities question the appropriate technologies to adopt for self-service: what is the most user friendly (but still robust) visualization tool? Are employees skilled enough to be able to use open-source tools such as R and Python?
Developing the right culture across the organization and federating siloed groups to maximize efficiency and spread knowledge is also a daunting, yet essential, element of self-service. How can you support growth without taking on too much, causing bottlenecks, and stifling efforts through over-management?
Defining Ownership and Process for Data Governance
When it comes to data governance, utilities struggle to determine the right points of ownership between business units and IT. When does the responsibility of the data fall from the hands of the business unit into IT, and vice versa? This problem is only aggravated by the adoption of new data and analytics technologies and tools.
Many member utilities find that as they become more advanced in analytics, technologies and systems architecture change, and a revision of data governance practices is necessary. Important to this process is understanding where and how data is being used, by both user and systems, defining and mapping the most important data, developing universal policies that appropriately govern it, and then actually embedding those processes into each business unit.
Enabling and Motivating Users
Visualization technology leaders such as Tableau and Power BI (Microsoft) have pushed out a lot of messages about developing self-service culture in the past couple of years, and rightfully so. Technology alone cannot motivate people to do something, nor necessarily do it correctly. This is something that utilities continually struggle to do, burdened by things like tool preference, tool literacy, actual or perceived challenges in working with data, and lack of structure to educate current and potential users. It takes a strong understanding of not only the organization as a whole, and how analytics are used or desired throughout, but also a clear vision of the desired future state. These are essential to map out an effective change management strategy that also enables culture to shift in the right direction.
Bringing together a community of utility professionals that are motivated to solve these challenges enables members to share and absorb advice and lessons. Currently our Analytics Architecture & Technologies Analytics Community is meeting monthly to discuss solutions that enable analytics self-service. Learn more about membership here.