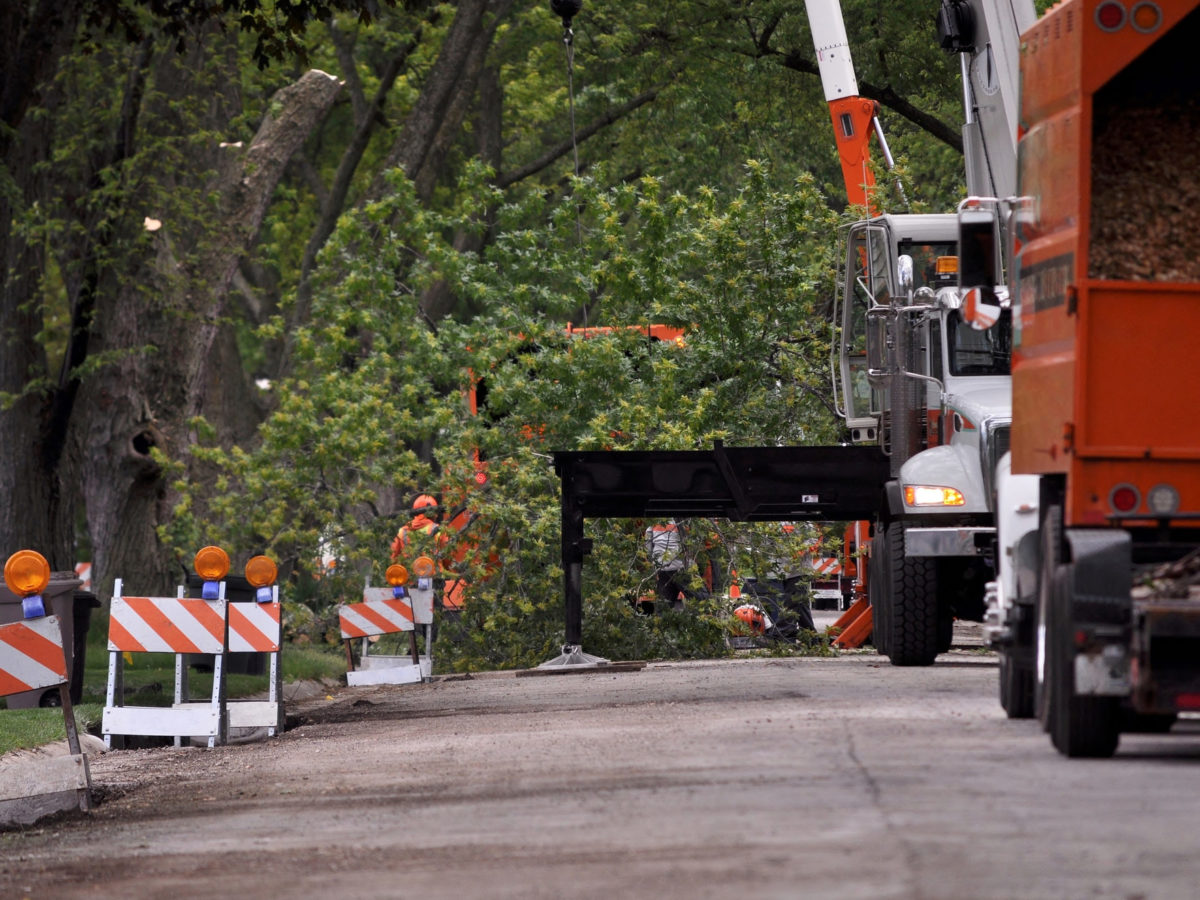
Hard work could reap big rewards in vegetation management.
The world of vegetation management (VM) has received significant press in 2019, and for good reason. In 2017 and 2018, a series of deadly wildfires struck the state of California. The 2019 season was particularly bad, forcing utility Pacific Gas and Electric to seek bankruptcy protection after incurring billions of dollars in wildfire-related liabilities. According to Elizaveta Malashenko, deputy executive director of the California Public Utilities Commission, utility ignitions account for 10% of Californian wildfires, and a primary cause of fires is vegetation contact with transmission lines. Malashenko also reported that Californian utilities spend about $1 billion annually on vegetation management.
California experiences exceptional circumstances and likely leads the world in VM costs; however, utilities in other jurisdictions also spend a considerable sum keeping vegetation away from power lines. In the UK this represents hundreds of millions of dollars.
Despite eating cash, VM is still a routine process
VM has been a critical area of focus for utilities and their regulators, and represents a significant chunk of a utility’s maintenance budget. Despite the many billions spent annually worldwide, utilities’ typical approach to VM is routine inspection. However, California’s problems may well accelerate the adoption of new techniques to improve the VM process. It was not so long ago that machine learning was touted as the latest technology to improve asset management, and it has since demonstrated its ability to reduce costs and unplanned stoppages. Many utilities are implementing some form of predictive maintenance in generation and transmission and distribution assets.
However, this shift from routine maintenance to condition-based and predictive maintenance has not occurred in the VM world. Running a predictive maintenance program on a wind turbine requires the collection of highly structured time-series data from sensors in the turbine. These streams can be analyzed for anomalies relatively easily, and historical data can be fed into a machine learning algorithm to improve the identification of problems before a major incident occurs and to filter out false positives.
Analytics-based VM relies on highly complex data
VM, however, relies on more varied, complex, and unstructured data, much of which is collected infrequently. To shift from routine inspections to a condition-based approach relies on far more sophisticated analysis than is currently used in the wind farm example.
The goal of analytics-based VM is to calculate (among other things) the likelihood of tree-fall in adverse weather conditions or when vegetation growth would contact power lines. To do this, utilities’ algorithms will rely on a lot of complex, unstructured data. Instead of time-series data, VM requires satellite and lidar images of power lines and rights of way. Handwritten inspection reports from the field could provide valuable insights. Then there is a vast range of secondary data that could help predict where vegetation could cause an outage: the growth rates of different tree species, land use data, microclimate data, weather forecasts, wind speeds, insect infestations, disease outbreaks, and many other factors.
Hard Work Could Reap Big Rewards
Given the amount of money utilities spend on VM, there is an opportunity for analytics to help improve the process. For larger utilities, there could be potential savings of tens of millions of dollars. However, this will not be easy. The complexity of the data involved requires important management, and the complexity of the analytics involved will surpass that of most utility data discovery projects. From the outset, utilities will have to pay close attention to model management, data management, and change management to make analytics-based VM a reality. Without strong information management, analytics-based VM could be another analytics project that fails to live up to its initial promise. Done well, analytics-based VM could reap millions in savings and improve grid reliability.
Stuart Ravens is a principal research analyst contributing to Navigant Research’s Digital Transformation service. Ravens has been an analyst for more than 20 years. For the past 10 years, his work has focused on the use of technology by utilities. He has played a lead role in the delivery of custom research and advisory work for many utilities, IT vendors, service providers, and renewables specialists.