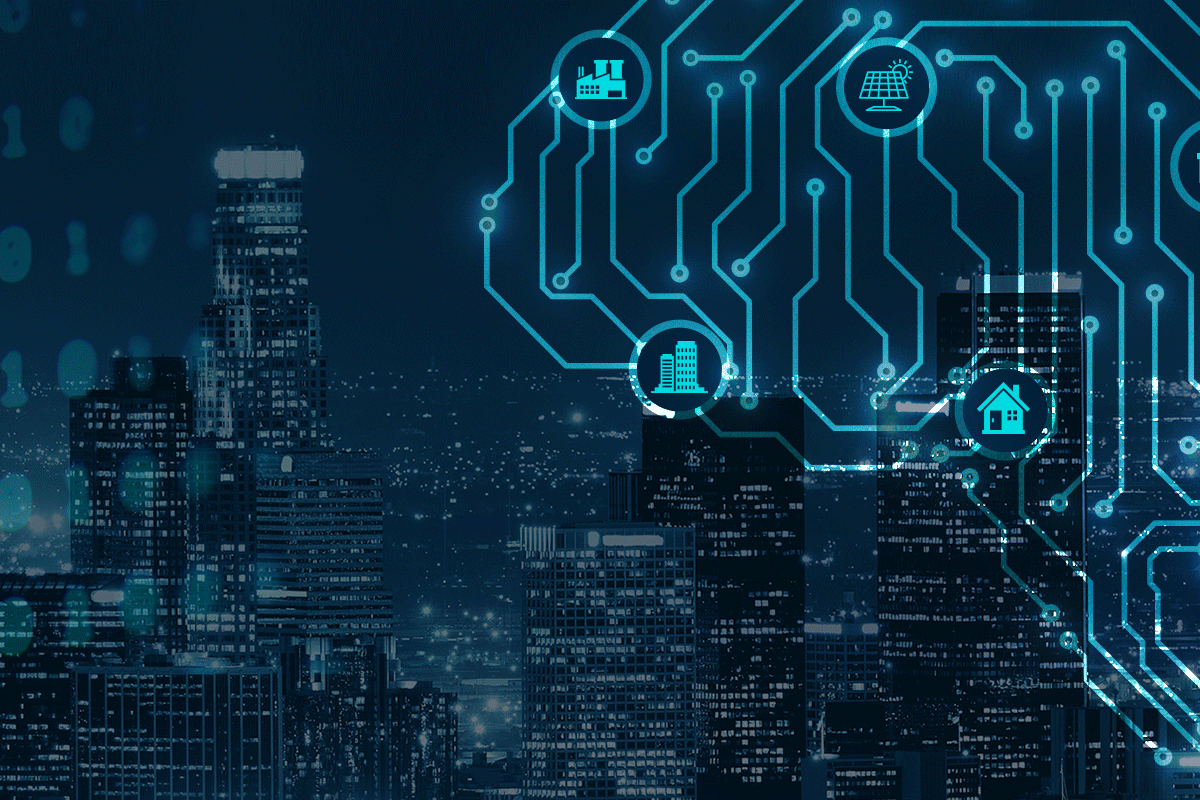
Utilities have seen the evidence; there is real value in using artificial intelligence (AI) to solve business problems. Artificial intelligence – encompassing machine learning, deep learning, natural language processing and image processing – has been successfully applied to benefit the customer and the grid at a number of utilities.
Despite high visibility, few understand what is involved in deploying AI to achieve results. That is about to change with the recent publication of the Department of Energy-funded study, “Voice of Experience: Artificial Intelligence – Demystify, Debunk and Deliver.”
The study was prepared by National Renewable Energy Laboratories (NREL) with the help of Utilities Analytics Institute (UAI). The effort included workshops at Utility Analytics Week 2019 (Salt River Project and Arizona Public Services) and CPS Energy, along with phone interviews. More than 60 utility analytics practitioners representing 40 utilities participated.
This guide hits home
Utilities, regardless of their maturity in adopting AI, will learn from the advice assembled from discussions with their peers.The report is easy to read and has a good combination of history, definitions (do you know the difference between supervised and reinforcement learning?), use cases and recommendations. Sprinkled throughout are quotes from utilities.
Even better are snip-its of how utilities have enabled AI:
- Salt River Project (SRP) –teaching and learning for the analytics community
- CPS Energy – analytics personas; access to data via representative state transfer (REST) APIs
- Duke Energy–the history behind the machine learning, artificial intelligence and deep learning lab (MADlab)
- Avangrid – analytics project-selection criteria
- DTE – experience providing self-service access
- Tacoma Power – analytics on the cloud
Readers have the opportunity to learn things they do not know and deepen insights into things they do know. The guide is helpful to utilities that are just getting started and those already on their way. Here are a couple of items that stood out.
Operationalization is not as easy as you think
By now, quite a few utilities have experimented with advanced analytics (including predictive, prescriptive and AI). The first step is applying AI to the data and validating results. One of the hardest parts comes next – operationalization. What is learned may lead to departure from traditional business processes. That rarely is easy for the organization to accept. It’s even harder to “automate” the learning into action. For example, if AI discovers that a storm is likely to hit a certain area that has vulnerable utility poles, how does that connect to creating a work order and dispatching workers to inspect the poles prior to the storm?
According to one practitioner, when moving from Proof of Concept (POC) into production, models often need to be tweaked and altered to function properly in the new environment. Once into production, subsequent updates to the product will be needed, based on building out planned features or user feedback.
Operationalization relies on cross functional teams with skills in project management, data science, data engineering and application development. Knowledge of the business is critical to the team. Also required is trust in the data and a basic understanding of the model workings and underlying assumptions.
It doesn’t have to be perfect
The guide gives permission for AI projects to be less than perfect. A lot of time often is spent on tweaking the models. Instead, the recommendation is to aim for improvement, not perfection. Given the nature of AI, models will improve over time with additional data and iterations.
The other message is that it is OK to fail–not that it is OK to fail operationally, but from an analytics project standpoint. Failure often is necessary to get to a better approach.
“The traditional project trajectory for most utilities involves a set of outcomes or completion points and is highly schedule-oriented. Participants in the Voice of Experience: Artificial Intelligence project emphasized how important it is for utilities to adopt a much more flexible, experimental attitude toward AI projects,” according to the guide. The good news is there are many ways that the cost of failure can be reduced – streamlining resources, time boxing efforts and adoption of Agile development.
Try UAI out
The introduction to the guide promises to “provide information that might not be accessible elsewhere— the kind you might get from talking to a colleague at a neighboring utility.” That promise was kept, in large part, due to the participation of UAI. The “birds of a feather” quality of UAI works well to keep utility analytics practitioners up to date on what peers (working from the trenches) have learned about the people, process and technology of embedding analytics in their organizations. Give us a try!