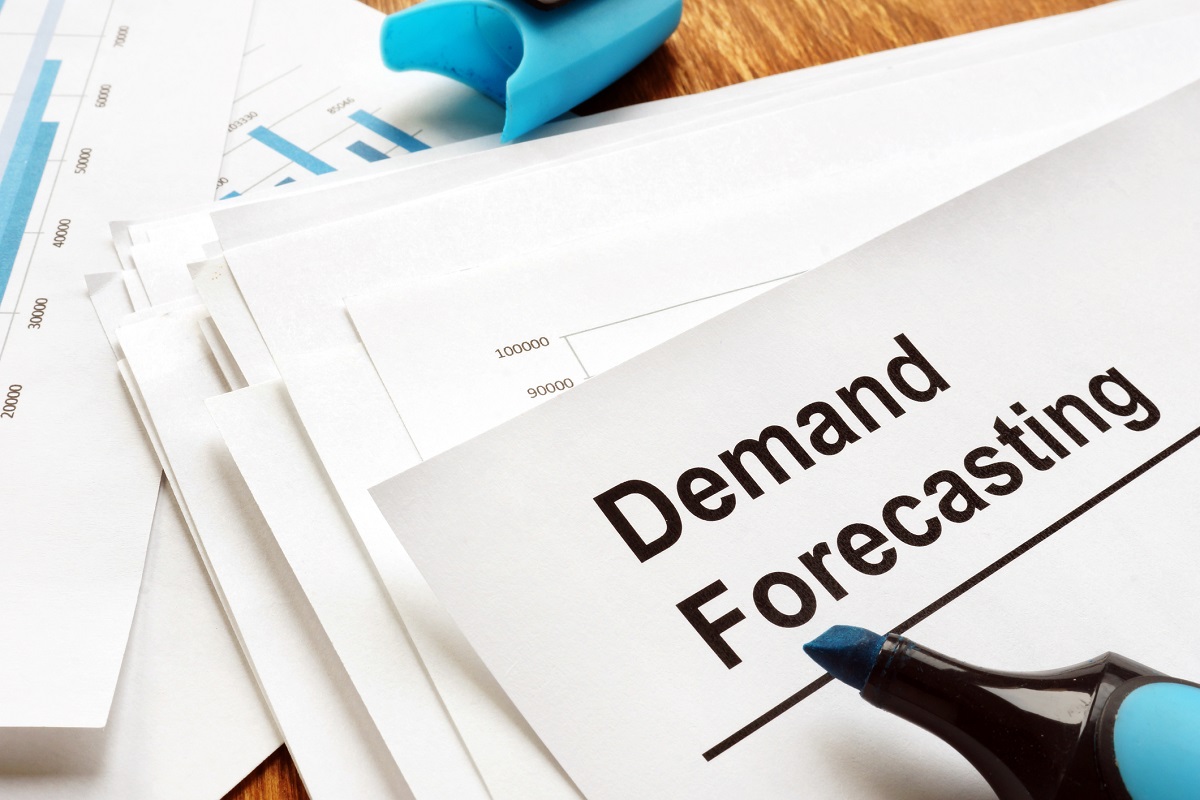
February Data Science Community Meeting
Estimating Electricity-Elasticity of GRP for Long-Term Demand Forecasting
Purpose
February’s Data Science Community Meeting featured Alex Elder, PhD, Data Scientist for The Energy Authority (TEA) speaking about estimating electricity-elasticity of GRP for long-term demand forecasting.
When it comes to long-term forecasting, data science tools are valuable but problem solving patterns differ for different contexts. For instance, historical data from the past twenty or thirty years may not serve as an accurate representation of what the economic environment will be like the in the future. This leads to sampling and stationary issues in long-term forecasting. The goal of this talk is to bridge the gap between regional utilities and macro-economic trends.
Summary
During this presentation, Alex ties electricity elasticity of GRP (gross regional product) to economic growth. The electricity elasticity of GRP is the precent change in electricity consumption in a given region or county that is associated with 1% increase in the economic output. This requires forecasting electricity demand or electrical load to understand changes in consumption.
When trying to predict variations in a region’s electricity demand or electrical load over long periods of time, variations in economic output or economic growth will have a larger impact over longer timescales because the economic changes are measurable. The timescales in question are days, years, and decades. Economic drivers increase in importance for longer timescales.
One area that indicates historical data will not accurately represent the future are societal trends that impact how people consume and conserve electricity. These trends substantially change consumption and conservation behavior from what it was in the past. For example, one of these societal trends is electric vehicles (EVs). Regulatory changes over time also invalidate historical data for forecasting.
Another data issue is limited sample size. For instance, when trying to build a model of electrical demand based on economic drivers over a period of time, yearly samples of economic data points are too small of a sample size to use.
Cross sectional analysis is the solution to quantitative long-term forecasts. In estimating electricity elasticity of GRP for long-term demand forecasting, the cross-sectional analysis compares geography, sample size, technological regimes, and regulatory regimes. The best data sources for cross sectional analysis are U.S EIA for service entity footprints and county borders; Woods and Poole for county-level economic and demographic data; and NOAA, weather underground, or vendor subscription for weather data. Merging the data and getting it into a format that is comparable is a technical challenge, but some tools can help overcome accomplish this. Multiple use cases were used to showcase solutions to this challenge.
Load per county is calculated and plotted against the economic growth per county on a log scale. The slope of that line is the electricity elasticity. Electricity elasticity is critical for an accurate long-term demand forecast. The biggest challenges to calculating electricity elasticity are limited data sets and unknown future societal and regulatory trends. Cross sectional analysis is the most effective tool for calculating long-term forecasts.
Data Science Community Members can watch the full recording here
Not a UAI member? Request to join: https://utilityanalytics.com/join/