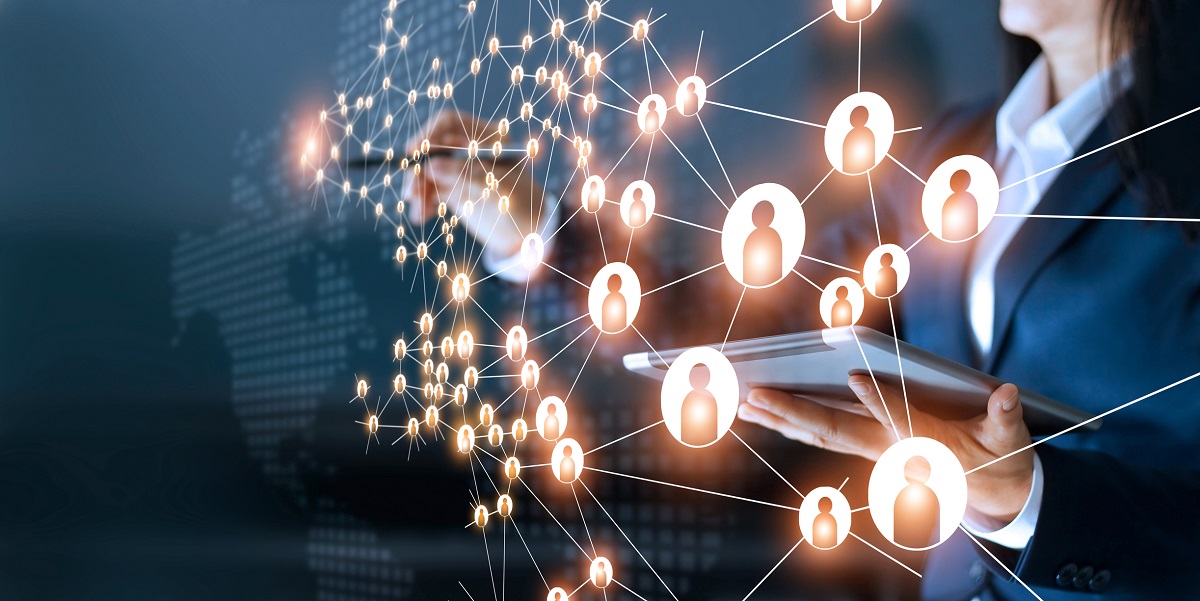
Blending customer-centricity and utility priorities is the art of portfolio optimization, but doing so can be perfectly scientific.
In the first two articles of this series, I detailed how utilities can 1) use data science to break down program silos to better serve customers and 2) turn up the volume on their data science efforts to understand each customer as an “audience of one,” enabling them to improve individual program performance.
In this article, I build on the tactics described and successes outlined in those first two pieces to move the needle on something every utility craves – unleashing data science to move beyond improved program performance (which is in itself a desirable outcome) to true portfolio optimization via more surgical customer engagement and resource acquisition.
Beyond the Basics: Portfolio Optimization
An electric utility with hundreds of thousands or millions of customers may literally have dozens of programs to engage them. The goal is to create efficiencies through these programs that benefit both parties; for example, lowering energy costs and demands on the grid, increasing the use of renewables, and increasing customer satisfaction.
Achieving these goals is easier said than done. Doing so holistically across a portfolio, so that one program’s gain is not another’s loss, has proven to be next to impossible.
That is, before the advent of data science. Fortunately for utilities, the tools – and know-how to apply them – are now available to extend individual program gains across an entire family of offerings.
To do so effectively, utilities must recognize two distinct types of data-driven optimization: customer-centric and product-centric. From a portfolio perspective, customer-centric optimization is about getting the right combination of offerings – in a single bundle or separately over time – to the customer. Doing so successfully avoids overwhelming the customer with too many choices (remember, they are customers, not energy PhDs) and comes across as personalized and thoughtful: Why yes, how did you know I was thinking of buying an EV, installing solar panels, and/or would be willing to pre-cool my home on the hottest days?
From a utility perspective, however, those tailored customer-centric offerings better make great business sense. Too often utilities operate with their ‘program blinders’ on, marketing to customers depending on which siloed product offer is currently being marketed vs. which one is ‘best’ for a given customer and, by extension, the utility itself. By ‘best,’ we mean the offering that will save the customer the most money, the utility the most energy, and create the most trust and satisfaction.
Know the Score
Blending customer-centricity and utility priorities is the art of portfolio optimization, but doing so can be perfectly scientific. It starts with scoring. Eventually, that scoring can lead to an even bigger and better outcome – designing programs from the outset with the right customers in mind, resulting in a portfolio that can effectively serve all customers over time to optimize customer lifetime value.
Crunching the data to assign customers a ‘propensity score’ is not what I am talking about here.
That path, while important, has become so well-trodden. It is a bit of a cliché in our industry, and not the catalyzing agent for the kind of optimization which utilities so actively seek. Part of the problem with standard propensity scoring is that answering “who is most likely” to act in a certain way, as in accepting a particular offer, only gets you so far.
Consider the following example.
A utility is offering two programs for load shifting. One is a behavior-based, time-of-use, demand response program where the customer must take proactive measures once notified of an upcoming event. A highly motivated, actively engaged customer in this program, one who enjoys the challenge of reducing usage in advance of the event by finding every conceivable way to do so, will be the one who saves the most money by participating in it.
But that isn’t for everyone. That’s why the utility also offers an alternative demand response program where load is shifted by the utility through automated approaches such as the auto-cycling of the customer’s air conditioning. A program like this can work great for someone who wants to be green, but isn’t such an enthusiast that they’d make the kids do their homework by candlelight.
I’m exaggerating a bit, but you get the point. These are two very different kinds of customers, and it is critical that the utility be able to tell them apart. Now, a typical propensity score assigned to both of these types of customers would show that each has a high likelihood of saying yes to participating in a load shifting program. However, utilities need something more granular. They need to make sure they aren’t sending the auto-cycling, smart thermostat offer to the customer (like the first one above) who would do so much more to maximize energy savings, because both parties will be leaving money, and energy, on the table.
So how can utilities achieve this granularity? They need to aim higher, using data science to do more than answer which customers should be targeted for a particular program. Today, data science can enable a ‘many to many’ computation that answers the question, “How can I best engage all of my customers across all of my programs?”
This is the good stuff.
Taking it to ‘11’
Let’s say a particular utility had 10 offerings in its portfolio. In a product-centric optimization, 10 analyses would be run to determine the best performance per program. In a customer-centric optimization, an 11th analysis would be run spanning the first 10, creating an optimized mix that determines the best strategy – i.e. the best set of offers to each customer – so the utility gets the biggest bang for its buck in terms of overall value across all its programs.
‘Value’ is the key word here.
As we tap into thousands of customer attributes beyond just basic demographics of age, income, and education to really hone in on each customer as an ‘audience of one,’ we can score them according the to the unique value they will provide a given program. Will he or she be that eager-beaver demand-response zealot, or someone who went in with the best of intentions but isn’t going to act on any of the requests? Put another way, will they be a great participator or a dud?
This value-based scoring combined with making that extra, ‘11th’ cross-portfolio analysis creates the first data-science backed, and AI-enhanced, opportunity for utilities to create personalized, multi-program offers optimized for value generation and customer satisfaction.
No Longer Just ‘Check the Box’
What I’m describing is a breakthrough, and we’re just on the cusp of it. The data science is continuing to improve as are the data sources, models and AI tools, including machine learning. While I am very excited about the win/win/win for customers, the environment, and utilities that I’ve been describing, I’m even more excited about the operational seismic shift it can enable.
Face it: The reason most utility programs operated in silos in the first place is because they were thought of as “check-the-box” offerings. For example, take a Smart Thermostat program that calculates it needs 100,000 average customers to hit the targeted load-shift goal. Once that goal was set, utilities typically outsourced the acquisition of Smart Thermostat customers and were blind to whether “good” or “bad” customers were being enrolled. It didn’t matter, as long as the program checked the box on the 100,000 target. This was a quantity game, not one about how customers would actually perform in the program or where they were served on the grid.
Fast-forward to today, and utilities are beginning to depend on these behind-the-meter resources to serve as non-wires alternatives with the same consistency as traditional grid assets. Power plants are being replaced by distributed energy resources and demand response, meaning these resources have to be counted on the same way a power plant could. An enormous amount of engineering and effort goes into traditional grid planning to ensure the right resources will be available to meet the expected energy demands, so why would a utility want to trust that “it will all just average out” when it comes to behind-the-meter resources?
This is no longer a check-the-box exercise.
And guess what? Maybe rather than depending on 100,000 ‘average’ customers to get the desired result, the same resource could be provided more efficiently by 30,000 of the best customers who are going to be dependable performers and are located in the right part of the grid. This type of efficiency would work wonders at the local level, too. For a utility facing a localized grid constraint, wouldn’t it be nice to call on behind-the-meter resources, whether solar, battery, DR or EE, and to use data-driven insights to count on those resources with the same consistency and reliability as traditional grid equipment rather than physically upsizing each substation to meet that occasional constraint?
This is the smarter, nimbler grid we’ve been dreaming about for some time now. With data science in its bloodstream, that grid is about to awaken to its full potential.
For more information, please visit E Source’s Data Science page HERE!
Tom Martin is Managing Director of Product for E Source Data Science.