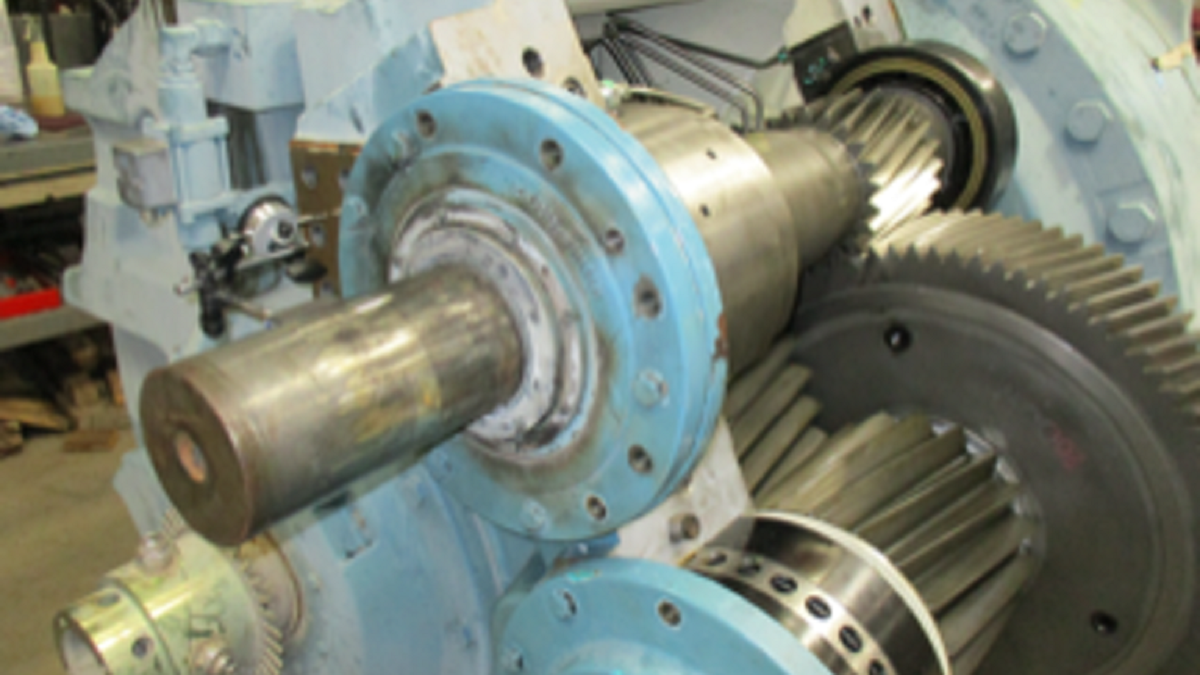
EPRI has been working with utilities in the United States to digitalize wind assets and develop a physics-based machine-learning hybrid model to identify gearbox damage and extend the life of gearboxes.
Wind turbine gearbox replacement cost can be as much as US$350,000. But EPRI’s physics-based AI early damage detection model, tested on WEC Energy Group, Southern Company and other utility’s wind fleets, may reduce repair cost to US$15,000-US$70,000.
As utilities work to support decarbonization goals, and incorporate or maintain more renewables into their portfolios, the reliability of those sources becomes vital to grid sustainability. So, when a major wind turbine component – like a gearbox – prematurely fails, production is lost, downtime can be prolonged and utilities’ operation and maintenance (O&M) costs increase.
In 2020, wind energy generation worldwide was 730 GW. It is predicted to reach 1455 GW by 2030 and 2434 GW by 2050, according to the 2020 International Renewable Energy Agency Global Renewables Outlook: Energy Transformation 2050.
With deployment of more wind turbines worldwide, R&D activities are increasingly important to ensuring the long-term reliability of turbine components, including the wind turbine gearbox. The gearbox converts low-speed rotations received from blades into higher speeds required by generators for electricity production (Figure 1).
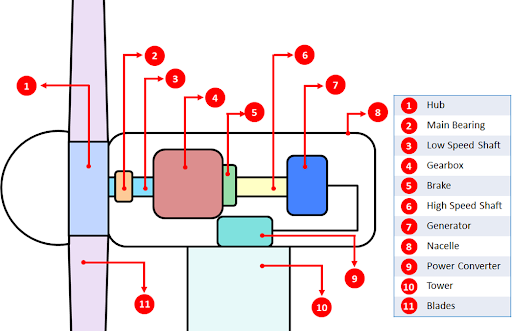
Figure 1: Wind turbine major components and their location
Electric Power Research Institute (EPRI) data and research show that the current gearbox cumulative failure rate during 20 years of operation is estimated to be in the range of 30% (best case scenario) to 70% (worst case scenario). The annual failure rate of gearboxes between 1-10 years of age is 0.8% to 2.3%, with an industry average of 1.6% (Figure 2).
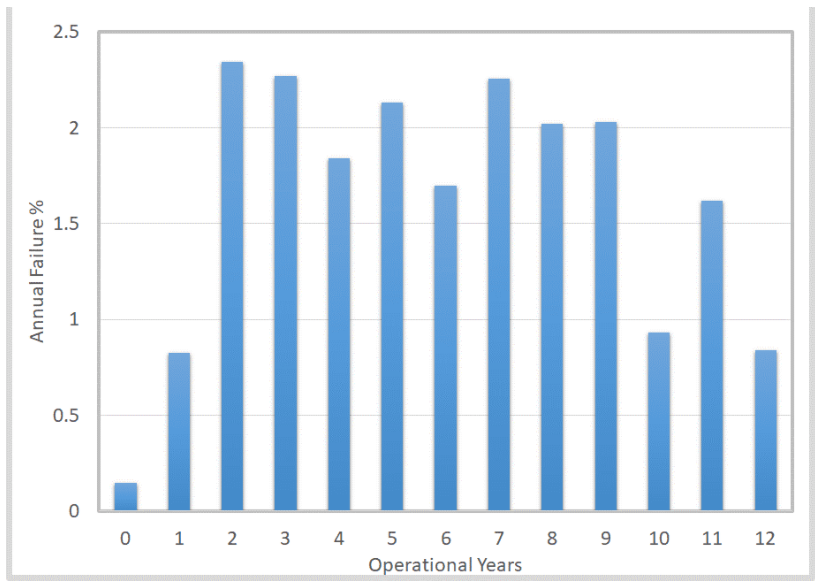
Figure 2: Wind turbine gearbox annual failure rate vs. operational years
Engineers and operators are constantly searching for ways to avoid failures and the high costs associated with them – a full gearbox (Figure 3) replacement can cost as much as US$350,000. But if a damaged bearing within a gearbox is identified early, the repair may only cost US$15,000-US$70,000.
EPRI has been working with utilities in the U.S. to digitalize wind assets and develop a physics-based machine-learning hybrid model to identify gearbox damage in its early stages and extend the life of gearboxes. Initially, physics-based models were used to detect failures. These models use equations to analyze gearbox material microstructure and properties for damage but are only about 60% accurate and cannot model and simulate a full gearbox system, its components, and their associated failure modes.
Combining machine learning with physics-based models for the wind turbines adds historic failure information and macro factors into the equation, such as wind speed, temperature, pressure, real-time power generation, etc. This brings a higher level of confidence in predicting the probability of gearbox damage and improves overall model accuracy.
EPRI researched and developed a physics-based and machine learning hybrid model solution after reviewing 10 different methods. In collaboration with utilities and operators, EPRI then successfully tested these early damage detection models not only on gearboxes, but also on main bearings and generators.
The physics-based machine learning hybrid model increased damage probability accuracy from 60 to 80%.
EPRI has been working with operators to support implementing these hybrid models at their monitoring and diagnostic centers as well as documenting millions of dollars in savings to date across several utilities.
“Participating in EPRI projects has allowed WEC to adopt wind turbine performance and health monitoring practices in-house which has led to substantial avoided capital costs,” said Cody Craig, asset manager for wind & solar at WEC Energy Group. “We are now able to proactively obtain notifications across our wind fleet that lead to low-cost repairs rather than running the equipment to catastrophic failure which is substantially more expensive to replace.”
Physical human data collection and validation might require a turbine operator to review 80 to 90 gearboxes per wind farm, each with 5 to 6 different bearing suppliers. Machine learning eliminates the need for those countless hours of resource time.
It is also less expensive to build the machine learning model than expanding the physics-based validation, which requires more in-person evaluation of gearboxes, and specimen comparisons.
“Innovative data analytics will grow in importance as wind resources are more widely deployed across the U.S. energy fleet,” said Steve Baxley, renewable, storage and distributed generation R&D manager for Southern Company. “The learnings from EPRI’s research show there are distinct advantages and cost benefits with the adoption of new monitoring techniques and data analysis, which can significantly improve wind turbine performance and O&M activities.”
EPRI’s hybrid model research has supported wind turbine operators in optimizing O&M actions and reducing high-cost repairs by identifying damage in its early stages and providing 2-7 months lead time.
EPRI is also assisting operators in improving budgeting and forecasting decisions, inventory management and resource planning, assist with component supplier selection and upgrades, and ultimately increase life extensions on turbines.
This hybrid AI model can be applied to multiple components across the industry, beyond wind turbines. EPRI is working on updating the model with more data, testing more advanced methods, and increasing accuracy across the model.
“This work is being used now by multiple utilities in the U.S. to inform O&M strategies, and we anticipate an uptick in international adoption as well,” said Neva Espinoza, EPRI’s vice-president of energy supply and low-carbon resources. “Enabling technologies like this AI model are an important aspect of the energy transformation as utilities consider ways to operate more flexibly and reliably in a decarbonized energy system. This AI model’s projections will enable operators to prioritize maintenance optimization and better estimate future efficiencies and costs, improving the overall operation of wind fleets.”