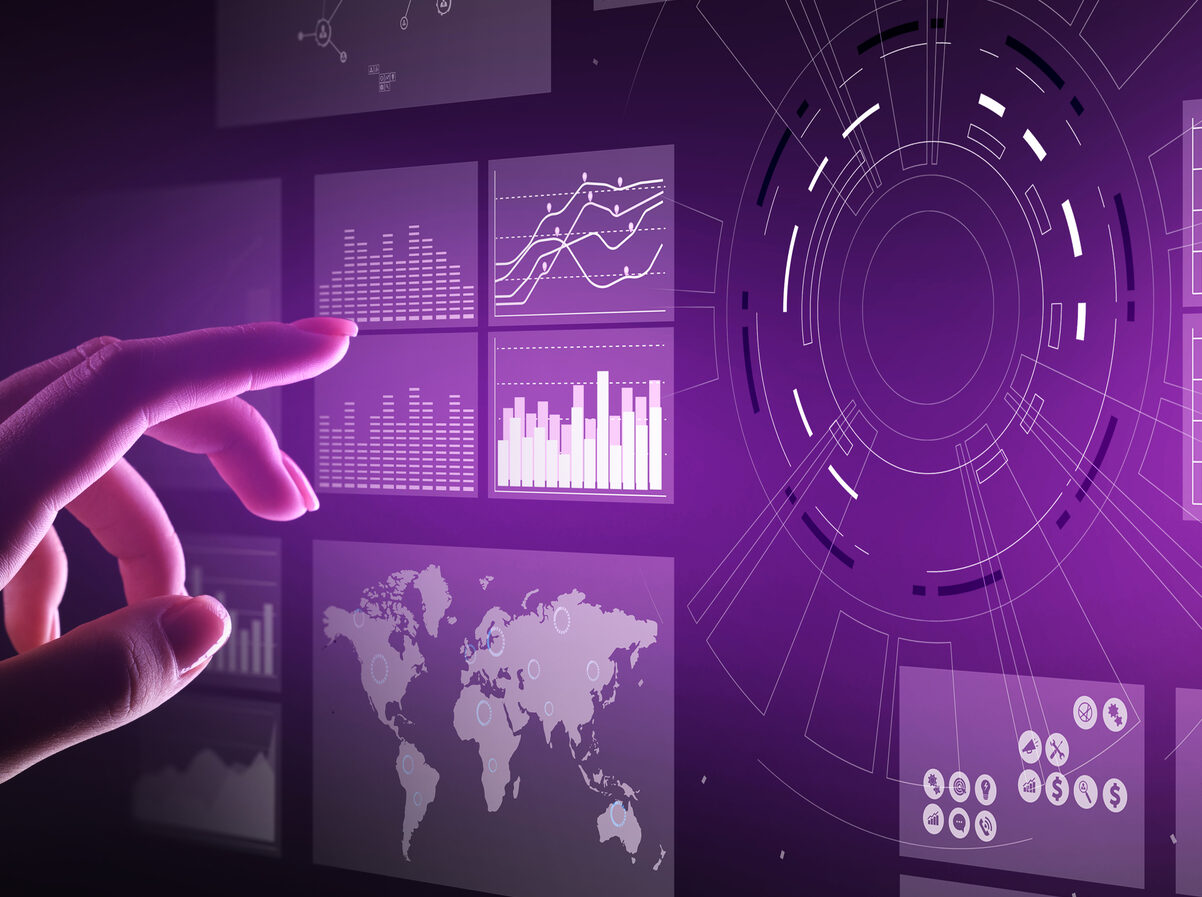
In early March, the Department of Energy (DOE) and the Environmental Protection Agency (EPA) agreed to collectively monitor and consult on what they called “electric sector resource adequacy and operational reliability (together, reliability) at a time of significant dynamism in the electric power sector.” That dynamic environment is the everyday reality of a modern electric utility. As the government gets deeper into sharing data to support electric reliability, though, utilities can do more data exploration of their own to change the dynamic.
Utilities’ systems are producing a bounty of data but, for the most part, it is an under-utilized resource—and that represents a missed opportunity. When data is properly captured, cleansed and understood, utilities can capitalize on this in frequently untapped ways. In short, getting ahead of potential issues reduces the range of costs attached to them—failure prediction leads to asset preservation, leads to lower capital expenditure. That’s good news in general, but especially so with transformer supply shortages looming. Data makes the difference.
Applying analytics to data begins with awareness. That means using the data coming off the electrical system to get a clear picture of potential catastrophic events, such as overloaded circuit damaging equipment causing outages, then using this insight to identify asset health concerns and prioritize the appropriate response. Capturing the whole picture—and the ability to drill down into the specifics—is the first challenge. The second is to get updated data frequently enough to effectively monitor changes to the system. From there, the ability to respond and predict begins to expand.
Propensity to failure isn’t a new concept, but technology is allowing new levels of insight into the topic. Real-time processing has become an impactful way to, in essence, measure streaming data against historical data to identify anomalies and generate alerts. This is based on a constantly learning and evolving analytical model that can recognize and predict failure patterns while they are still affordable to fix. This ultimately makes for happy equipment, happy budget and happy customers (and possibly happy regulators, to boot).
Multi-variate streaming data (processed by a gradient boost model) presents real-time reports on potential transformer overload. Drilling down to examine how much and how often a specific transformer is exceeding its capacity, then comparing that data to a map of the asset’s health over time, allows for a detailed analysis of the transformer’s behavior. This can be zoomed up to the substation level to analyze the same types of data, for example, enabling the identification of certain transformer types that demonstrate shared trends. Adding geo-location to these visualizations provides further insight into likely root causes and possible wider impacts of asset failure.
Detecting previously hidden patterns in data starts with successful aggregation of data, but it ends with faster, better troubleshooting; prescriptive maintenance strategies; and reduced downtime. These techniques can be applied across a broad range of data streaming off utility assets and, ultimately, drive down costs by targeting the leading indicators of failure and taking timely preventative action.
Where these processes get really interesting is when the data reveals issues that might otherwise go unnoticed. We recently worked with a customer to apply the technology to fleet management of a variety of wind turbines. This quickly unearthed a peculiar sensor signature that pointed to a probable coolant leak. When maintenance crews investigated, it turned out to be a hard-to-spot leak that, if it worsened, could have resulted in a lengthy outage.
Comparing the data from that turbine to others in the fleet identified several similar problems—with the potential to cause failure within the year—that could be promptly addressed. These kinds of outcomes encourage utilities to expand their data exploration and refine their approach to maintaining a strong system, and devise new, creative ways to apply the technology to the industry’s many complex systems.
The “dynamic context” described by DOE and EPA in their new memorandum of understanding doesn’t need to be articulated to the utility industry. What could be better articulated, as data analytics capabilities continue to expand, is the most direct and cost-effective path to prevent that dynamism from having an unexpected and outsized impact on reliability and resiliency.
Learn more about these techniques and their many applications for the utility industry by reading our white paper “Five Ways Asset Situational Intelligence Enables Utility Resilience.”