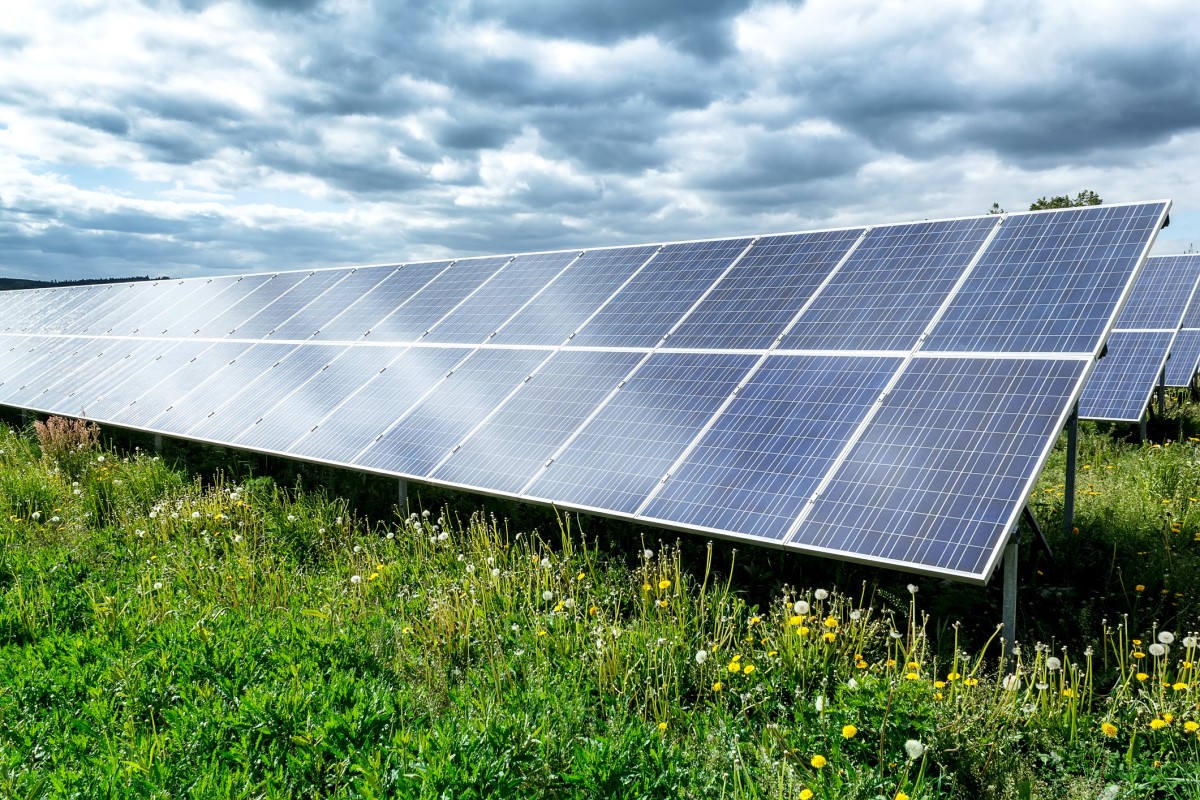
Today’s energy industry is undergoing profound changes in response to the demands of climate change mitigation. To improve their operations, optimize resources, and enhance customer experiences, utilities are increasingly turning to data-driven solutions and adopting cutting-edge methods to tackle the increasing complexity posed by the green transition. From the realm of quantum computing algorithms, Quantum Machine Learning (QML), a fusion of quantum computing and artificial intelligence, holds the potential to transform the industry by enabling the efficient utilization of vast amounts of data.
The Data Challenge
Utilities companies collect immense volumes of data every day, including information on consumption patterns, equipment health, and environmental conditions. Analyzing this data efficiently and effectively is a significant challenge, especially when dealing with traditional computing methods. As an example, modern smart grids face challenges when it comes to scalability and efficient processing of the sheer amount of data flowing from a vast network of sensors, communication infrastructure, energy prosumers and connected devices. The complex nature of utility systems demands a more sophisticated approach than traditional solutions can provide, and that’s where QML comes into play.
Quantum Machine Learning Opportunities
QML combines quantum computing and machine learning to solve complex problems that can be practically impossible for classical computers. Quantum computing uses the principles of quantum mechanics to process and analyze information in ways that far exceed the capabilities of classical computers. By applying machine learning algorithms to quantum computing, utilities companies can unlock insights from their data that were previously hidden as well as improve their predictive models.
The field of applicability of QML to the sector is broad. Some examples are:
- Enhanced data analysis
- Predictive maintenance
- Optimization of energy distribution
- Renewable energy integration
Forecasting Renewable Energy Production
One promising use case for QML is the real-time prediction of energy production by a wind or solar farm. Renewable energy production forecasting is crucial for the cost-efficient integration of renewable resources in energy networks but at the same time it is extremely hard due to its variability. In a real-market scenario, these forecasts are re-computed several times a day with the latest data available to adjust energy market offers.
Traditional Machine Learning methods usually struggle with this type of requirement, due to the large amount of heterogeneous data, which is often incomplete. Indeed, classical ML algorithms are inefficiently trained with this type of data and are often unable to capture intricate correlations and new dynamic trends in the renewables market. On top of that, traditional algorithms cannot handle incomplete data very well, leading to inefficient solutions and predictions.
QML algorithms are specifically designed to tackle big data more efficiently and are able to find hidden correlations between available data. This allows the algorithms to generate on the fly more accurate real-time predictions with incomplete data.
Climate Change and Weather Forecasts
Temperature has been historically one of the most monitored data by the utilities sector: cold temperatures would push up gas and energy demand, which in turn would affect prices. However, with the increasing contribution of renewables to the energy mix, the importance of other weather variables has significantly increased. Indeed, energy production has become more dependent and correlated to cloud coverage and wind conditions to mention just a few. Furthermore, historical data are expected to be less reliable due to the weather changes attributed to climate change and the emergence of unseen patterns calls for more accurate forecasts.
The mathematical basis of computer models for weather forecasts relies on the numerical resolution of complex Partial Differential Equations (PDE), the so-called Navier-Stokes equations. Solving this type of equations requires high amounts of computational power and could greatly benefit from quantum computing.
Recent papers 1,2,3 indicate that quantum computers could improve classical methods by leveraging the power of quantum algorithms, which are more suited to efficiently simulating the dynamics of complex and intricate systems.
QML has the potential to generate important advancements in the area: with the improvement of GPUs and computational power, machine learning models have been successfully used to approximate the solutions for this type of equations. However, training these models requires time and many resources. QML has the ability to process multiple states concurrently, which can reduce the training time considerably. In addition, QML operations rely on various laws of quantum physics that are more apt to simulate the dynamics of fluid systems.
Putting Data to Work with QML
QML has the potential to revolutionize the utilities industry by harnessing the full power of data. With the ability to process vast datasets more efficiently and accurately as well as identify hidden correlations, utilities companies can use QML to make informed decisions, optimize resources, reduce costs, and enhance customer experiences. While there are challenges to overcome, the benefits of QML are significant, making it a promising tool to navigate the complex and data-rich landscape of utilities operations.
References
- Tennie, F., and T. N. Palmer, 2023: Quantum Computers for Weather and Climate Prediction: The Good, the Bad, and the Noisy, Bulletin of the American Meteorological Society, 104, E488–E500.
- Harrow, A., Hassidim, A. and Lloyd, S. Quantum Algorithm for Linear Systems of Equations, Physical Review of Letters. 103 (15) 2009.
- Lloyd, S. et al. Quantum algorithm for nonlinear differential equations, arXiv:2011.06571.
About the Authors
Multiverse’s Chief Operating Officer Gianni Del Bimbo is a quantum machine learning specialist and responsible for designing, implementing and overseeing day-to-day operations to drive the company’s strategy. Gianni holds a MSc in Condensed Matter Physics from the Ludwig Maximilian University (LMU) of Munich and a BSc in Physics and Astrophysics from the University of Florence.
Aser Cortines is a director of engineering at Multiverse Computing and leads a team that works on projects in finance, energy optimization and machine learning. Aser holds a PhD in Probability Theory from the University of Paris and an MSc in applied mathematics from l’École Polytechnique de Paris.
What is UAI?
Utility Analytics Institute (UAI) is the only community enabling utility transformation through analytics.