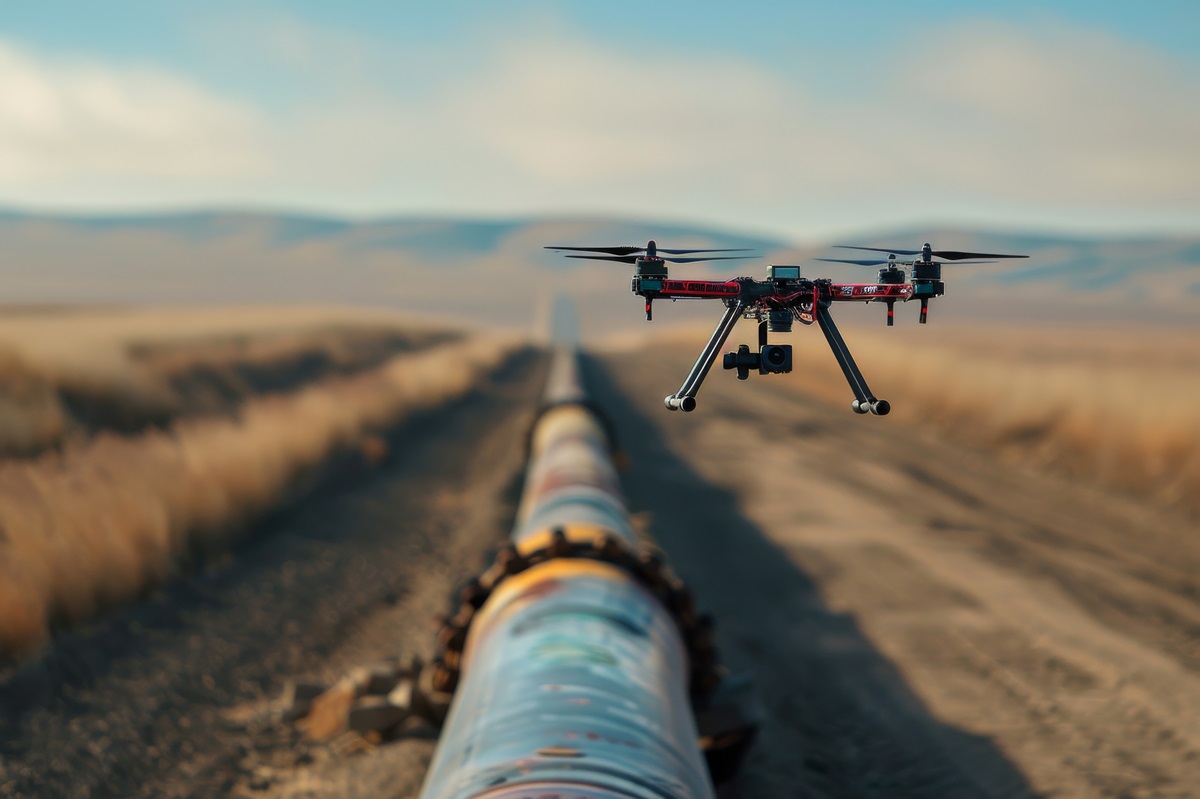
Facing the challenges of an aging infrastructure, budgetary constraints, and escalating compliance demands, more utilities are realizing the operational advantages of asset image analytics. By analyzing images from drones, satellites, LiDAR, and fixed cameras, utility providers gain powerful data assets that drive strategic decisions regarding asset management, vegetation management, emergency response, and more. With each truck roll costing as much as $1,000, image analytics offer a viable alternative to manual inspection, with the added benefit of reducing risk to employees in evaluating assets in hard-to-reach or hazardous areas.
The lifeblood of asset image analytics is data—data that is rapidly growing both in volume and complexity. Individual images can be as large as 8,000 megapixels, and a single drone can capture more than 100 images per hour when inspecting power lines. To adapt to this growing complexity, utilities need to establish a solid data foundation for an image analytics program that is up to the task of enhancing operational efficiency and reliability.
Beyond “big data”
In addition to the images themselves, utilities must address escalating amounts of metadata to feed their analytics platforms. This includes “natural” metadata, such as geospatial location, date and time, etc., as well as what I call “manufactured” metadata, which includes image recognition output, tags from other data sources such as asset maps, and standardized metadata schemas to enable faster search and retrieval.
Large-scale data from asset images also demands special attention to storage and integration requirements. Retrieving relevant data from a huge number of image files requires careful, consistent organization and tagging. Incoming images must be split, tagged, and stored securely while maintaining accessibility and integration with other systems.
Building a robust foundation for image analytics
To ensure image analytics deliver valuable insights—and remain scalable to accommodate ever-growing volumes of information—utilities need a framework that enables effective management of their image data.
An effective data platform incorporates the key characteristics of effective image data:
Searchable
Data should be searchable by attributes such as asset ID, asset location, or image date.
Clean
Not all images are equally beneficial; only images that deliver value should be retained to avoid clogging the system with unusable items.
Intelligently tagged
Image tags should be logical and consistent with those used in other systems.
Accessible via a centralized image repository
Utilities should create a single source of truth by integrating with existing utility systems and applications to avoid data silos and provide seamless access to image data through RESTful APIs.
Consumable
Image data should be tagged and indexed in a way that allows other applications to integrate it seamlessly.
Extensible
Image data platforms should be capable of bringing in data from third-party data sets such as asset maps and weather records.
360-degree views of assets
Image data should incorporate all relevant angles of an asset to enable comprehensive assessments.
Design for the future, but begin with the present
While it can be tempting to jump right in and start building a fully fledged asset image analytics platform, utilities are well advised to use their current state as a starting point—to conduct a thorough assessment of their present data ecosystem and compare it to the next logical phase of maturity. By following a phased approach, the organization can take the first steps towards an effective asset image analytics program without committing resources to capabilities their systems are not yet ready to support.
Setting the stage for effective asset image analytics
Collecting data from drones, LiDAR, satellites, and other sources is just the beginning of an effective asset image analytics program. To effectively transform raw image data into actionable insights, utilities first need a foundation in the form of an accessible, scalable platform.
By prioritizing good data hygiene and systems interoperability, utility providers can set the stage for image analytics that are capable of driving strategically sound decisions on asset management, vegetation management, emergency response, and other critical areas. As the maturity of its image analytics capabilities progresses, the utility becomes increasingly capable of keeping up with the increasing complexity of its data—and the demands of an ever-evolving utilities industry.
This article was written with the assistance of artificial intelligence.
About the author
Mick Wagner is responsible for leading the Advanced Analytics Practice to exceed client expectations, develop innovative solutions, and achieve organizational growth via the Logic20/20 Advanced Analytics offerings in Modern Data Platforms, Strategic Insights, and Data Science Excellence. Mick has over 15 years of data analytics consulting experience across the full BI lifecycle—defining data strategy and architecture, designing metadata-driven ETL frameworks, leading large teams in custom software development, developing data analytics, and defining KPIs across Fortune 100 and nonprofit clients. He strives to provide simple solutions to complex problems by leveraging proven technical solutions and management methodologies.
is a sponsor of
and a member of