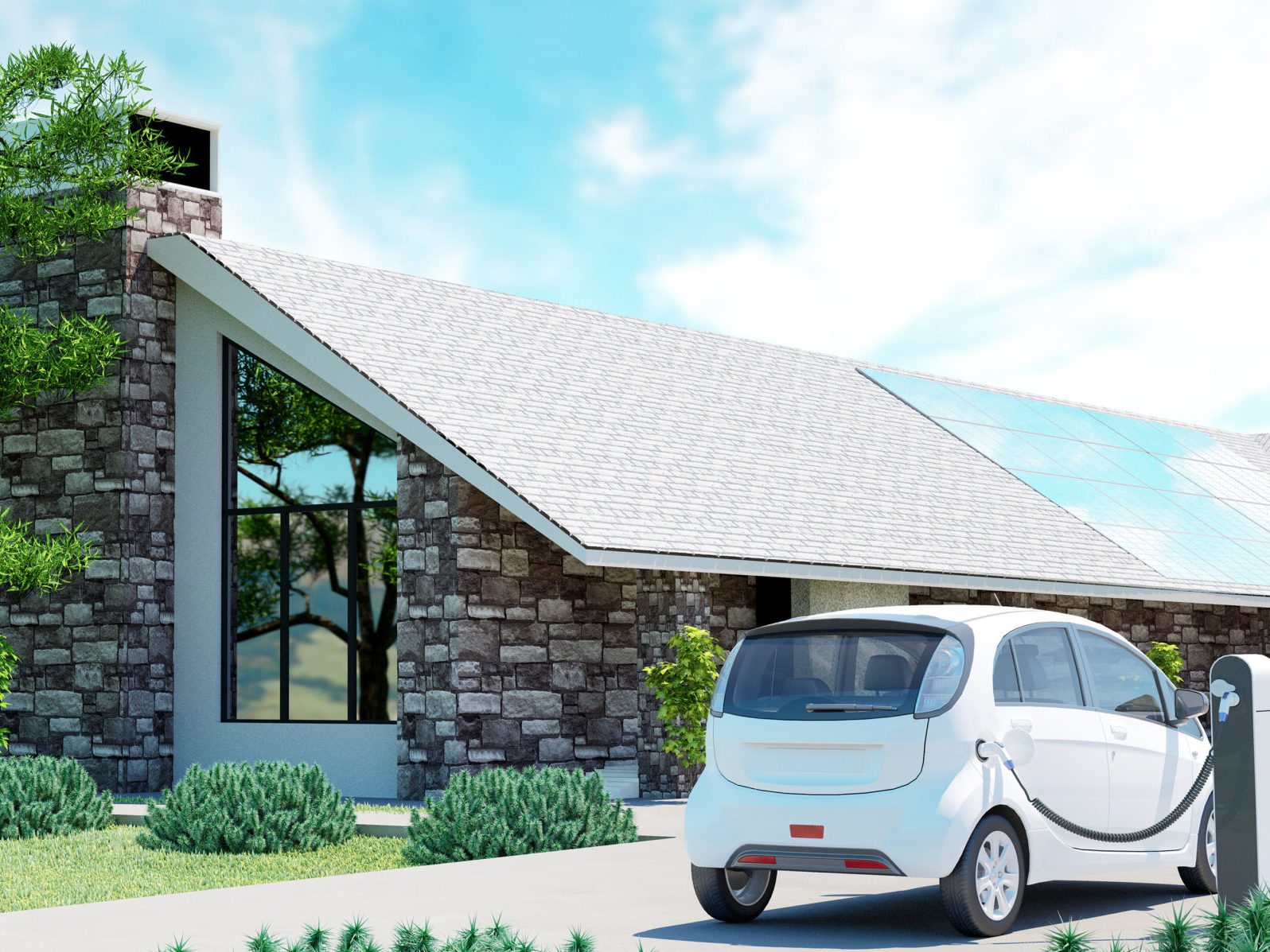
The penetration of distributed energy resources (DERs) is growing rapidly. Forward looking utilities are engaged in planning exercises to learn how DERs will impact their business. Concerned utilities want to understand the impact of DERs on revenue (minus for DGs, plus for EVs) and grid stability. What investments are needed? What least-cost approaches will be acceptable to regulators? This sounds like a job for analytics, especially forecasting, simulation and optimization. The big question is whether or not analytics are ready for this.
A case study at Sacramento Municipal Utilities District (SMUD)
A recent SEPA report, Beyond the Meter: Planning the Distributed Energy Future Volume II , discusses how one utility, SMUD, tackled this challenge. The report set out a step-wise approach to planning:
- Customer DER Potential and Adoption Forecast
- Distribution Impact Modelling
- Bulk Level Impact Modelling
- Financial Impact Modeling
The first step was to forecast which individual customers will adopt DERs (and what type of DER), where and when they will adopt them, and how adoption might change their usage behavior. SMUD used NREL’s Systems Advisor model, excel and proprietary models to determine the technical and economic potential of their forecast, which included energy efficiency, CHP, PV, EVs and energy storage. Since the report, SMUD has been working with a vendor to create a more comprehensive forecast, using machine learning to improve adoption models.
Distribution impact modeling provided insight into potential grid performance, helping SMUD to understand how DERs may affect the grid at the feeder level, as well as downstream of the feeder level. By modeling power flow for a subset of substations using a commercially available grid simulation platform, the utility could better understand the most cost effective ways to bolster their system.
Then there was the question of how high-DER penetration would offset the need for traditional generation sources (for SMUD this was hydro power and natural gas generation). SMUD used a commercially available production cost modeling tool to simulate the impact on bulk-level energy, capacity, dispatch and emissions.
Lastly, financial impact modeling got to the question that near and dear to the hearts of many: what is the monetary value of DERs at various locations on the grid? If SMUD wanted to encourage more DERs in constrained locations, what incentives or new rate structures would motivate customers to install them?
Connecting the dots is not straightforward
The process was largely instructive, giving SMUD a good understanding of the implications of DER on their business. However, there was a lot of heavy lifting involved. Each step involved multiple analyses and there was no easy way to feed the results from one step to another.
Other utilities are also seeing limitations in the tools available for DER planning. There are a lot of missing pieces; many models are proprietary, Excel-based, and not easily replicable, for example. Regulators do not always agree on which methodologies should be used. These and other factors put many utilities in a position where they are forced to rely on professional services firms that work with different proprietary and non-proprietary engineering and economic models to pull everything together.
For now, the critical gaps are in adoption forecasting and distribution planning. DER load profiles are no longer predictable, making traditional load forecasting obsolete. Although a handful of research organizations are stepping up to the challenge. (note EPRI’s response to the utilities’ demand for completely new methodologies, models and approaches for load forecasting), these models remain nascent.
For distribution planning, the attention needs to go to the secondary circuit level and in some instances, the sub-hourly level. Residential customer adoption of DERs requires utilities to address the need for added capacity (with EVs) or mitigation of voltage, back-feed or power quality issues (with intermittent generation).
The big question is whether analytics maturity will develop fast enough to help utilities prepare. In Europe, forecasts have fallen short. Utilities there are handling unexpected DER impacts by shoring up situational awareness capabilities of distribution operating systems.
Are DER-heavy utilities being held captive by technology limitations? How can next-generation analytics like machine learning and artificial intelligence be used alongside engineering and economic models to leapfrog efforts? What do you think?