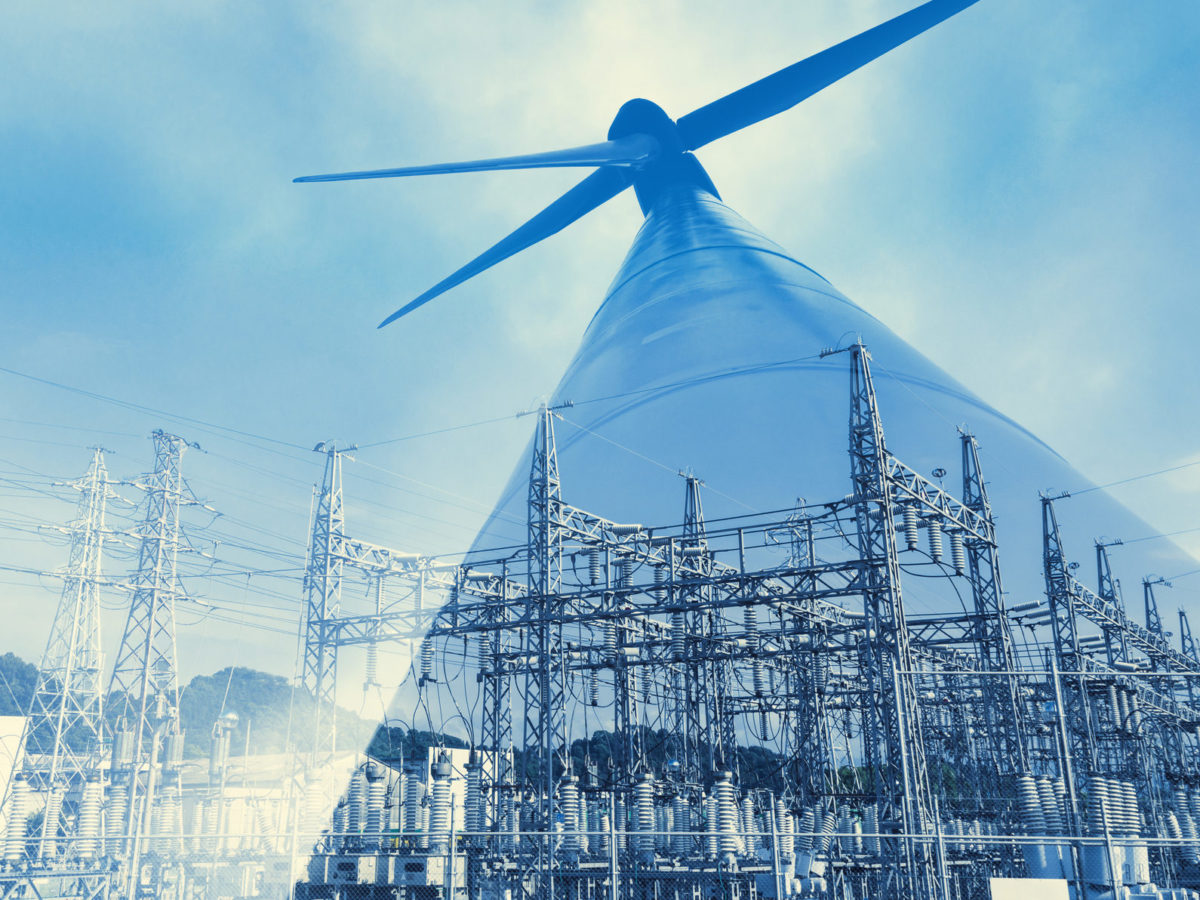
Digital twins hold promise, but not without a massive data headache
The digital twin market is exploding—some say to the tune of $15.66 billion by 2023, at a CAGR of almost 40%. Utilities are among many asset-intensive industries poised to benefit richly from these technologies.
Digital twins are virtual models of assets that can be used to gain both real-time and predictive insights on performance. As a platform, they can live in the cloud, and significantly reduce costs and risks associated with construction, maintenance, and performance optimization strategies. They can also enable savings through process improvement and for utilities, open the doors for new business opportunities integrating distributed energy resources (DER) such as distributed solar PV and electric vehicles.
Digital twins can also be a valuable sandbox to test the performance of real-time and edge analytics. Utilities can “deploy” the most risk-intensive forms of analytics: predictive modeling, machine learning, and artificial intelligence, without taking on much actual risk. From mitigating skeptics’ evidence of analytical “fails”, to the loss of millions of dollars caused by perverse machine learning (ML) or artificial intelligence (AI) outcomes (when an algorithm literally “learns” something incorrectly based on a false positive or faulty goal identification, for example), the potential value of a functional digital twin cannot be underestimated.
Note that I said a functional digital twin. What I mean by that is a digital twin that performs like the real, live asset, meaning that it must have the data it needs to inform it to do so. This is often where the technology falls short.
There is always an issue with data
A digital twin provides a model of an asset primarily based on its initial 3D CAD model (or something similar). It is then layered with any relevant data gathered from the system, such as SCADA, sensor, meter, and any other IoT data that might impact it. The more data the digital twin has, the more accurately performance and potential outcomes can be modeled. Likewise, the complexity multiplies with as more data streams are integrated.
But what happens when a utility would like to operate with multiple digital twins, or in the case of the electric grid, model an entire network? It’s becoming more common for strategic assets to be supplied with a digital twin right out of the box. A utility can quickly find themselves in possession of hundreds, or even thousands, of digital twins—each being fed data from just as many systems and IoT devices. The complex task of accurately informing a single digital twin will continue to be amplified by integrating multiple digital twins in a network. The effects of bad data on such a system can easily be staggering.
Furthermore, it was not that long ago that smart meters were testing the bandwidth capabilities for utilities. A digital twin scenario for a single utility will require exponentially more data from the edge. Even with an abundance of sophisticated, low-cost edge computing technologies to perform more granular analysis, data will still need to be brought home to feed the model.
Additionally, there is a lack of technology that supports data management at the edge. Many of the necessary data management functions that are required to provide useful data still need to happen at a more centralized location. According to Ovum’s 2018 ICT survey for utilities, nearly 53% of utilities ranked data management as a significant challenge (a further 33% stated that it was somewhat challenging).
This is not to say that digital twin technologies will be immensely valuable to utilities, especially in an increasingly distributed energy future. It will just be crucial to front-end investments with data management considerations.
Lauren Callaway is a Senior Content Manager at Informa.