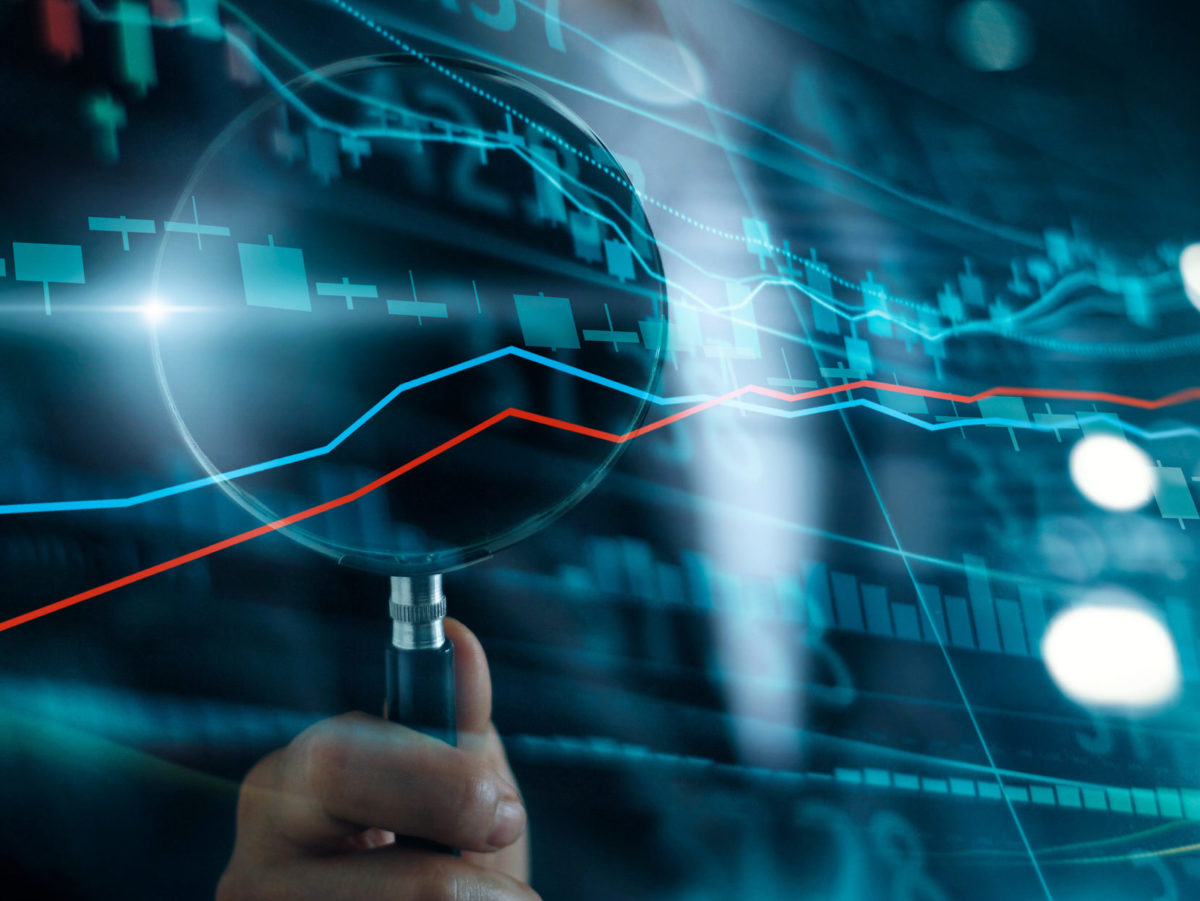
Modernization of the grid, digitization of the customer experience, and replacing back office infrastructure to support complex rate structures are just some of the factors influencing utility rate increases. Combine these with an economic slowdown in the US and utilities across the country are facing increased collections costs and bad debt. For those that don’t improve their collections’ activities, the additional costs will likely lead to additional rate increases, perpetuating an increasingly tense relationship with customers and low customer satisfaction.
How are utilities typically approaching collections? And more importantly, what role can predictive analytics play in reducing bad debt and collection costs at your utility regardless of economic conditions?
To help answer these questions, I recently spoke with industry experts Jody Allison, who recently served as Vice President of Transformation for Algonquin Power & Utilities Corp., and James Riley, a digital and analytics advisor to Sempra Utilities.
Historically, how have utilities differentiated between customers for credit and collections? And what are the limitations of such an approach?
JA: “Utilities have traditionally used two sources of information to differentiate between customers: external credit scores and internal credit scores. For new customers, utilities are initially reliant on external credit scores from providers such as Equifax, Experian, and TransUnion. Over time, as a pattern of payment behavior for a specific customer can be measured, the focus shifts to an internal credit score, or creditworthiness.”
JR: “I think we have all heard or read the phrase ‘past performance is no guarantee of future results,’ usually with respect to financial services. The problem with both external and internal credit scores is that they assume future results will indeed match past performance. A customer who has never had a problem paying their bills in the past is assumed to be low risk, whereas a customer who has had problems in the past is assumed to be high risk. It just isn’t that simple.
“While internal credit scores measure a customer’s behavior, specifically towards that utility, external credit scores are often less specific and reflect a customer’s behavior with respect to a variety of different products and services. These are blunt instruments upon which to base credit and collection strategies.”
How can predictive analytics help sharpen a utility’s credit and collection strategies as a key first step to reducing bad debt and collections costs?
JR: “A propensity-to-pay predictive model can rank all of a utility’s customers according to their likelihood to pay their debt, once in arrears. Such a model does not assume that past performance will predict future results but will take it into consideration. It is much more powerful.
“Let’s imagine a utility has two customers, A and B. Both customers have the same external credit score, and since moving into the utility’s territory have paid all their bills in full and on time. Historically, utilities would treat these customers identically. A propensity-to-pay model can tell the utility that Customer A will almost certainly pay if they fall into arrears, but that Customer B almost certainly won’t and will likely result in bad debt.”
JA: “How could a utility benefit from the example James just provided? Well, if I know that Customer A will almost certainly pay, then I will use gentle reminders, use low-cost channels, and won’t rush to roll a truck to disconnect them only to roll another truck to reconnect. By contrast, if I know Customer B likely won’t pay, then I will reach out quickly, my reminders will be very direct, and I will prioritize a truck roll sooner rather than later to minimize the likely bad debt.
“Most utilities already have the key ingredient for building a predictive model—historic data, and lots of it. By analyzing historic payment behavior, and perhaps combining it with external sources of data, it is possible to identify the characteristics that drive any customer’s propensity-to-pay score and use those characteristics to score and rank all customers irrespective of observed past behavior.”
Turning Knowledge into Action
Increased spending is exerting pressure on utilities to raise their rates. Combine this with an economic slowdown across the US, and utilities face the prospect of increased bad debt and escalating collections costs.
Traditionally utilities have differentiated their credit and collection strategies based upon internal and external credit scores, on the assumption that for each customer past performance will be an accurate predictor of future results. A propensity-to-pay model goes further by predicting how different customers will behave if they fall into arrears, even if they have never done so before. Understanding this is a key first step to helping utilities reduce bad debt and collection costs.
Now that you understand the benefits of predictive analytics and propensity-to-pay models, “Reducing Bad Debt & Collection Costs with Predictive Analytics: Part 2” will provide steps for building your own model and operationalizing it.