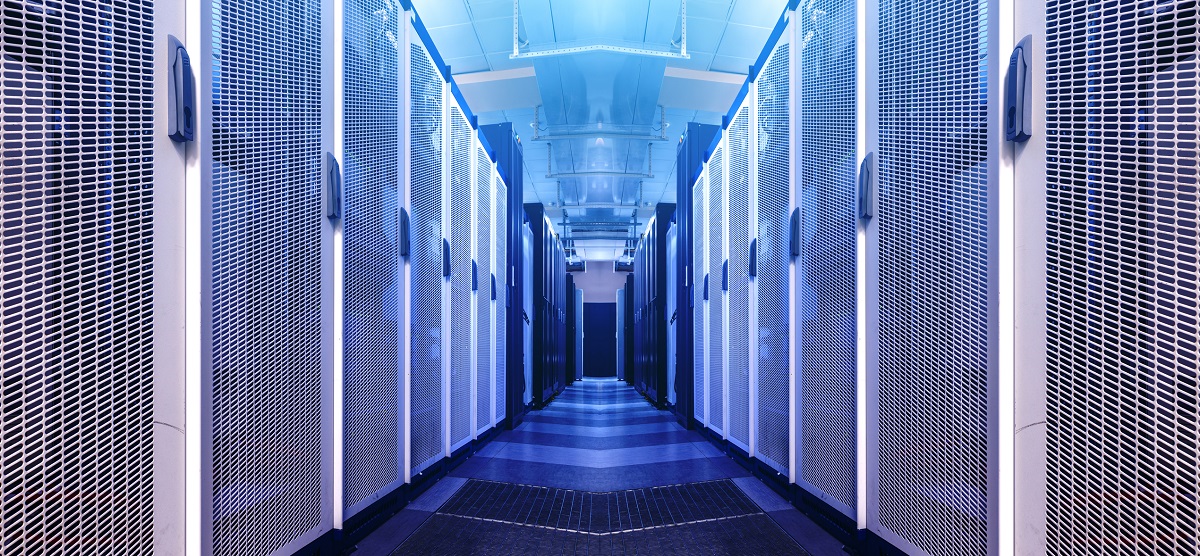
Connecting DWH to advanced analytics at scale requires a data fabric, not just data availability.
For industrial companies, the path to ultimate value from data liberation requires three crucial steps. Many organizations have already achieved step one: liberating data from siloed source systems and aggregating it in a traditional data warehouse (DWH). The bad news: Step two is far more difficult to achieve. The good news: The rewards for successfully taking it are correspondingly higher.
In today’s mature DWH market, progressive, data-driven organizations are actively utilizing data fabric solutions as a complement to existing DWH strategies. By using data fabric, organizations can liberate their data once again–lifting it from the pool of aggregation and turning it into contextualized knowledge to deliver on their ambitions for advanced analytics.
What is data fabric? And what sets it apart from data warehousing?
The two main pillars of data fabric are Context and Discovery. They define data fabric and make it distinctly different from and complementary to existing DWH.
- Data context is the sum of meaningful use, case supportive relationships within and across different data types and data artifacts. It is the result of data relationship mining and curation in a so-called contextualization pipeline. The process of adding context to data often is referred to as data contextualization or data fusion.
Prior to contextualization, data often is integrated from many source systems and co-located in a common data repository, similar to traditional DWH. Alternatively, data integration is virtualized through data federation, which avoids the need for data duplication and transfer. More recently, a hybrid approach has become common, especially for latency-sensitive Internet Of Things (IoT) data applications, where data aggregation and data synthesis must be performed close to the data.
What is the industrial status quo?
In power and utilities, digitalization efforts have long been limited to pilot projects, proofs of concept and case studies, with no large-scale operationalized projects. This is mainly due to outdated IT infrastructures that rely on legacy systems and only enable point-to-point integrations for application providers. These one-off solutions–which sometimes include limited digital twins–actually can complicate digitalization goals because the resulting projects are as siloed as the original data, making them impossible to scale and, therefore, costly to the point of wastefulness.
Complementing existing DWH solutions with data fabric has dramatically reduced costs, while simultaneously enabling scalability, speed of development and data openness throughout our many complex customer organizations.
- Data discovery is about making data effortlessly available to the right user in the right format. This always has been the goal of data and information architects. Discovery in B2C technology is instantaneous, autonomous and continuously self-learning. In other words, it’s far ahead of enterprise and IoT data discovery. But that’s where we’re going: shifting from active search to passive discovery based on personalized relevance.
Recently, the exponential increase in data volume, velocity and business value, coupled with the meteoric rise of low-code and citizen-data science programs, is making data discovery more important than ever before.
In the context of enterprise data management, enabling the right data to be easily discoverable relies on much the same recipe: the right metadata, labeling, linkages to other data, and data cataloging to make it readable by both machines and humans. Outdated manual metadata management is gradually being replaced by active, machine learning-supported metadata practices, used to discover and infer additional metadata from relationships and clustering.
This is why progressive organizations are seeking out data fabric solutions to complement their DWH strategies. Data fabric adds critical context and discovery to existing DWH data assets. In fact, complementing DWH with data fabric is the only way to push through all three steps to achieve true data liberation.
Leveraging a Data Fabric to Improve Use Case Efficiency
Now let’s further put data fabric in context with the data warehouse. Intended to address key challenges posed by data accessibility, scalability and efficiency, the data warehouse is undoubtedly an important part of the evolving technology stack for use case delivery. But it’s become clear that a few limitations remain unaddressed. While the data lake creates the environment for data reusability (through better accessibility of data from its source system as well as a way to adopt standards that enable scalability), it falls short of improving efficiency in the use case development process.
As you can see in the below diagram, while more data can be accessed in one place in the data warehouse, it still does not quite solve the contextualization that must occur before the data becomes useful for the business use case. As a result, data must be re-contextualized each time it is used in a use case, exposing a significant and manually taxing inefficiency.
Here’s where the data fabric comes into play as the means to 1) make it easier to contextualize data sets for the first time, 2) automatically contextualize new data into data models, 3) enable the reusability of these data models for new use cases.
As you can see, this next generation architecture is critical to keeping new use cases from requiring the same effort as the first proof of concept while reducing the IT burden and costs for maintaining multiple data solutions over the course of their lifecycles. The digital solution stack continues to evolve in practice and architecture, and both data warehouse and data fabric will continue to play an important, symbiotic role.
Gabe Prado is the senior director of product marketing for power and utilities at Cognite.