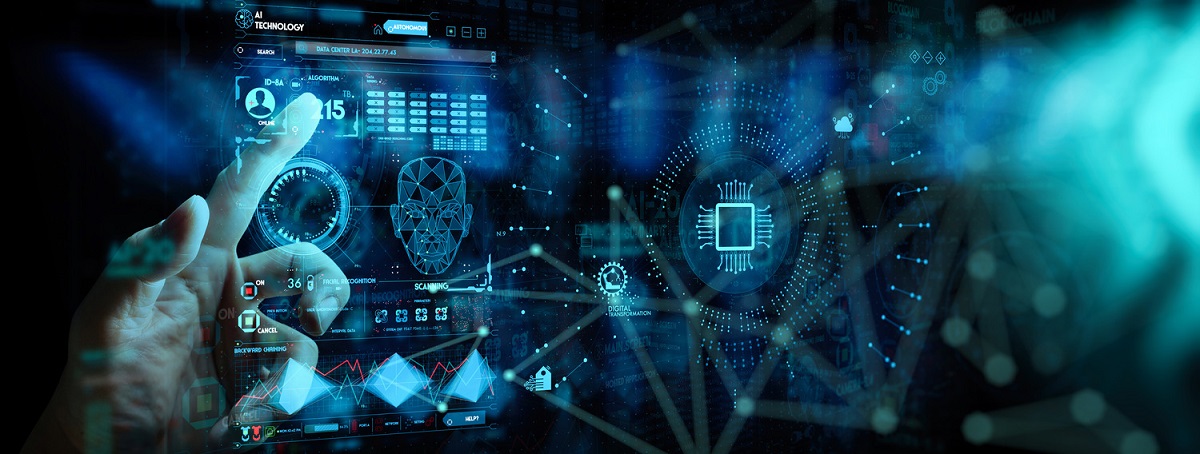
Rappahannock Electric Cooperative worked with Burns & McDonnell to simulate load flows in order to develop a digital model that would assist in understanding the correlations between loading, load flow and losses within the system.
Rappahannock Electric Cooperative (REC), Fredericksburg, Va. has long known that the kilowatt hours it purchases are not equal to the kilowatt hours it sells. Could this discrepancy be caused by system line losses or could other factors, such as theft, be the cause?
These questions led to a detailed line loss analytics project in partnership with Burns & McDonnell, an engineering and construction firm based in Kansas City, Mo. The goal was to gain an accurate and in-depth understanding of this persistent issue by creating several digital dashboards that would give REC employees the ability to maintain an easy-to-understand visual display of actual system performance in near real time.
Thanks to project results, the dashboards and related insights produced by this analytic journey are helping REC gain understanding of its system losses, generating cost reductions that will benefit members.
Building a Model
As with so many complex systems, building a digital model was crucial to understanding REC’s transmission and distribution system. The Burns & McDonnell team worked with REC to simulate load flows in order to develop a digital model that would assist in understanding the correlations between loading, load flow and losses within the system. The simulations are based on large volumes of data, which include loss reports, voltage values and precise loading ranges for each node.
As regularly refreshed data became available, it was analyzed and uploaded to visualization programs to assist with validation methods and provide feedback that would lead to improvements and efficiencies.
The team used computational platforms to derive key data associations within the model. This was necessary to manage the massive volume of data that was being fed into the model, and it helped yield insights into critical associations. The platforms were especially helpful in situations when actual data from circuits and other sources were not available.
Engineering and Data Science
With a system-level model in hand, the team turned to integrating several large and previously disjointed datasets. A full range of system operational and control data generated by the supervisory control and data acquisition (SCADA) system was a critical foundation. Additional data from automated metering infrastructure (AMI) and metering data management (MDM) systems were then overlaid to gain additional insights into a technical loss analysis that identified loading of the electrical infrastructure at specific periods. This provided a picture of the varying ranges of voltage flow over the distribution circuits system-wide.
The data science element of the project focused on providing the deeper insight needed to understand potential causes of system-level losses. It was understood, prior to the project, that a number of factors could be driving these losses. For this step, the team engaged in a deeper investigation using advanced analytics algorithms designed to explore the relationships between SCADA, AMI and MDM systems for detection of unmetered loads, losses from system conditions such as impedance, or possible energy theft.
The models built for the engineering analysis (to simulate the network) helped the data scientists gain understanding of customer profiles and the different circuits that fed power to certain large commercial customers or residential areas. Based on these models, algorithms were built to help analyze correlations.
Unusual Energy Usage
The essential question for the team was: What is driving the usage jumps? If AMI readings exceeded average levels of kilowatt-hour usage, it was a clue to dig deeper. If it was a residential meter, could the spike have been caused by electric vehicle charging? If commercial or industrial meters showed unusual power consumption, other possible explanations were explored.
This portion of the project involved reviewing the billing data from wholesale energy purchased at the transmission delivery point in comparison to cleansed retail billing data. This comparison was a key step in analyzing how much energy REC was losing on its system. From a billing perspective, the assumption was that unsold energy is considered a loss to REC.
Accounting for Unmetered Loads
Data generated by AMI and SCADA fed algorithms to identify locations where energy losses were occurring. The algorithm used AMI data generated for each circuit — along with power flow estimates from the engineering technical study — to create estimates of the data that would likely have been generated by SCADA systems. These loading estimates were incorporated into the algorithm to further refine the portion of energy load that was not accounted for by metering data. These estimates were derived by other methods as well, including AMI to phase matching, AMI to transformer associations, and other methods aimed at approximating the source SCADA levels.
It is typical for utilities to have contracts and rates for customers that set up monthly or other periodic billing cycles based on kilowatt-hours used, instead of energy consumption on an hourly basis. This factor was a challenge for this project because it required formulating a method to accurately estimate the losses and loads for given periods of time.
Actionable Insights
The project has provided REC with many actionable insights into the different functions of load and losses indicative of its system. This has resulted in alignment of core systems for a precision approach to prioritizing and reducing losses in the future.
The project involved stepping back for a macro view of some basic questions such as: What is the correlation between AMI and SCADA? What does the line loss look like? Ultimately, the goal is to make all the puzzle pieces fit so that a complete picture emerges.
Overall, the project provided a detailed understanding of how and when losses were occurring, as well as a granular view of system performance from delivery point, substation and circuit at various times that could provide clues to help solve the puzzle.
Those calculations are driving insights into many factors that will sustain prudent management of wholesale power costs at the cooperative electric utility for member-owners.
Eugene Hamrick is an Analytics Solutions Architect at Rappahannock Electric Cooperative. Carol Bogacz is a Lead Data Scientist at Burns & McDonnell.