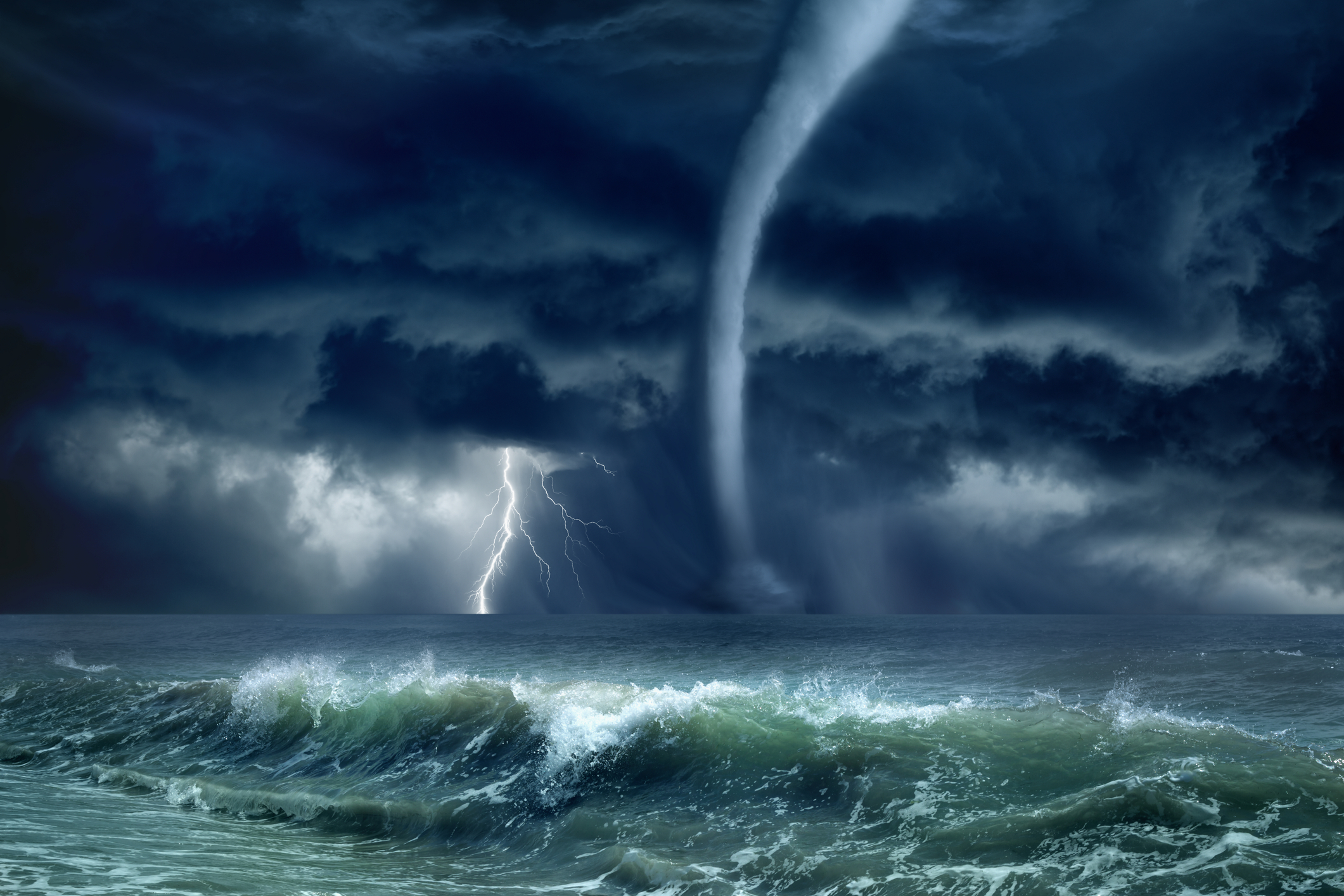
Four steps to enterprise data management for climate-challenged utilities
From megadroughts to rapidly intensifying hurricanes, tornado outbreaks and record-breaking temperatures, our public infrastructure is being stressed to the extremes of its original designs. The National Oceanic and Atmospheric Administration has been tracking billion-dollar-plus events since 1980 and reports that the past five years account for 34.4% of total weather disaster costs ($742 billion out of nearly $2.2 trillion), underscoring that extreme weather is not only getting more frequent but also more costly.
This fast-evolving landscape has made salient an overlooked aspect of 21st-century reality: our water, gas, electric and transportation infrastructure is vast, complex and fragile within an increasingly volatile environment. Effective use of data is critical to unlocking the decisions that protect companies, stakeholders, customers and communities. We know that a good solution involves being data-informed — the question is, how do we make it happen?
Data Identification, Collection & Curation
Before building or revamping an enterprise data management program, utilities can start by identifying what decisions need to be made. For example, an electric utility would like to identify anomalous mechanical failures of different conductor types based on unusual outage or repair frequencies. Data to collect for this analysis include a record of historical outages (including the cause category, location and duration) and repair records (including the work done, the component being serviced and the date completed). The details become even more complex when you consider nuances such as how an outage cause is classified (for example, the labels may have changed over time).
High-quality data curation is complex for any organization. Data must be clean and useable (for example, of high enough fidelity or refresh rate to capture physical and operating conditions), robust, and compatible with downstream systems such as risk and reliability models. For organizations that manage geographically dispersed assets, such as electrical transmission and distribution circuits, pipelines, and railways, accurate data collection is only the first step—and should encompass multiple data points for thousands of individual system components.
Quality Analysis, Clash Detection & Repair
Once organizational data is fully integrated, it must be cross-checked for inconsistencies and errors. This process is nearly impossible to accomplish with separate data streams. Whereas partial integration can afford manual opportunities, full integration enables automated capture and analysis for continuous review, correction and reporting. However, even utilities that achieve full integration rarely have a process in place to both identify anomalies (“clash detection”) and systematically trigger data record repair. This often is due to siloed lines of business that don’t trace data back to their original sources. Taking a technical view of how an error surfaced (whether through a construction crew, mapper, design engineer, etc.), what it may represent in the larger data ecosystem, and how it could impact decision-making can help prevent repeat occurrences and avoid erroneous assumptions.
Data Analytics & Visualization
With a fully integrated, rigorously “clash detected” and systematically repaired data ecosystem in hand, the analytics needed to empower operational and planning decisions can take place. For example, when evaluating the risk and reliability of a network of assets, there are three customizable categories to consider:
- Threat: The state of the asset relative to its age and environmental conditions, such as rate of corrosion, decay or mechanical wear.
- Hazard: The exposure of the asset to environmental events such as wind, earthquakes, flooding and extreme temperatures.
- Consequence: A quantitative measure of the effect of an event—for example, the number of customers that will lose power if a particular electrical transmission structure fails on a particular circuit.
In broad strokes, the output of these models should offer valuable insights into asset resilience, system resistance and inspection planning. Since risk can vary with environmental conditions on any given day, so too should the models that inform decision-makers.
Act & Evolve
Risk- and reliability-driven choices will be prioritized naturally by the level of threat, hazard and consequence, but they also must be weighed against the feasibility of different choices. A transportation agency considering a road expansion on the cusp of wildfire season, for example, will have to decide whether the project can be completed without increasing risk or whether it should be delayed. A strong enterprise data management system can be leveraged not only to make better critical decisions but also to continue evolving. For example, a model that influences behavior may become less predictive as behavior changes; hence, it must be adaptable as the system it seeks to measure and predict also changes.