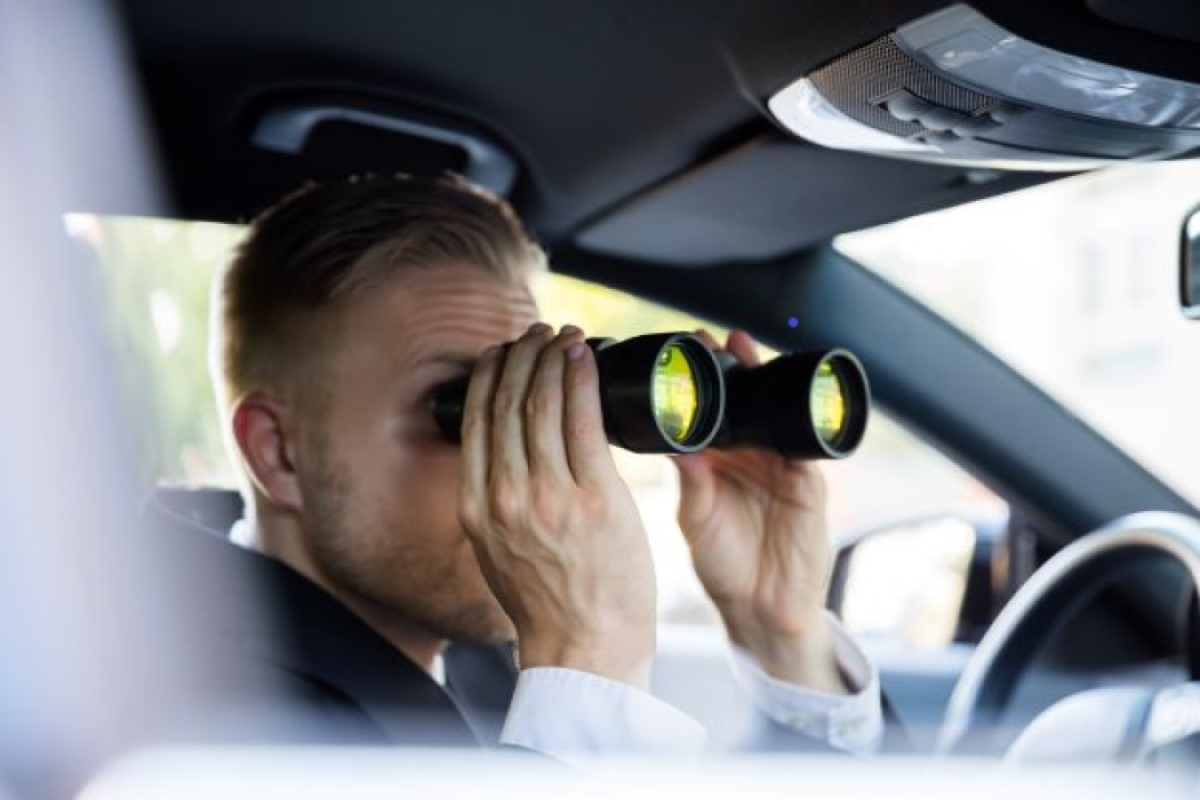
Zondits speaks with a forecasting specialist about modeling EV adoption to optimize infrastructure deployment. Walter Schaefer is a Senior Engineer at DNV with eight years of experience in electric mobility and transportation, demand side management, and distributed energy technologies. In addition to his residential EV adoption forecasting work, he is currently leading several innovative EV efforts, including designing and evaluating managed charging pilots designed to shift charging off-peak via financial incentives and behavioral mechanisms, analyzing the utilization of utility-funded charger networks, and modeling the economic and operational feasibility of fleet electrification.
The electric vehicle (EV) industry is growing rapidly and there are many important questions that need to be answered about how such growth will impact the power grid and how utilities can accommodate and manage the load. Further, with the federal funding bills that have been approved, there not only is a mandate, but a unique opportunity to optimize the deployment of new infrastructure to support the EV industry. A primary question is how to best plan for the deployment of EV infrastructure effectively and in a way that is impactful and equitable.
Hi Walter, thanks for taking the time to talk with Zondits. We understand you are involved in EV Adoption Forecasting (EVAF) – what is it and can it help optimize infrastructure deployment?
EV Adoption Forecasting is an approach to modeling the underlying drivers of EV adoption at a geographically granular level in order to build up insights about when and where EVs will start to arrive, or increase in numbers, in our neighborhoods (and on the grid). We’ve all seen the market change rapidly in the last 1-2 years, with several new EV models becoming available and a sea of change in customer awareness and interest, and we expect the market to change even more as the result of the tax incentives and domestic supply chain development efforts included in the Inflation Reduction Act. As such, it is critical to forecast these changing EV adoption dynamics so that utilities and their regulators can make smart decisions around growing and/or updating their EV program offerings, planning and prioritizing distribution system upgrades, and addressing equitable community deployment of public EV charging infrastructure. It is imperative that utilities have accurate and detailed forecast insights to support their decision-making processes and help streamline the transition to EVs.
In your opinion, what is the best approach to forecast EV adoption?
Customized agent-based modeling (ABM) is a sophisticated approach that I believe is a great method for forecasting EV adoption for a number of reasons.
- It incorporates customer preference and demographic data to triangulate on the main drivers of EV adoption. This can be very powerful if you set up the model appropriately to incorporate customers’ awareness of, interest in, and preferences regarding EVs, and also include the demographic landscape at the neighborhood level.
- This approach also allows us to effectively model the impact of peer effects, the fact that when people see and interact with EVs in their day-to-day lives, their own likelihood to adopt increases. This ability to model agent interactions and evolution is unique to agent-based modeling.
- The geographic granularity is another powerful aspect of ABM. We know EV adoption will not be uniform everywhere, whether that’s between different neighborhoods within the same city or in different states. It’s highly correlated with wealth/income, education, location, and one’s level of awareness of/experience with EVs. Agent-based modeling allows us to capture and forecast these adoption trends that we know will vary across different areas and different populations.
The forecast is also probabilistic, which helps capture the somewhat random nature of who is willing and able to buy or lease an EV and when. It’s also flexible, allowing us to run multiple adoption scenarios (such as high, mid, and low possibilities).
That’s informative – are there other forecasting approaches besides ABM?
Absolutely – there are several different options out there, including system dynamics models and simpler extrapolation-based approaches.
- The system dynamics (SD) approach relies on fairly complex models of future EV costs and benefits, the incentive and policy landscape, and customer price sensitivity. We see an exciting overlap with this approach and ABM. The SD approach can be used to generate forecasts across large geographies and long timescales. For example, forecasting the entire North American vehicle market out to 2050 or 2080. To do that, one needs to build out detailed cost and technology curves, which are also of value in ABM. While a powerful tool in its own right, a system dynamics model is not designed to capture the probabilistic decision-making process of individual consumers with diverse backgrounds, which is an area in which the ABM excels.
- Extrapolation forecasting methods rely on recent historical data to make estimates about the future. This approach may require potentially costly input data and, more importantly, is limited by the levels of EV adoption we’ve seen in the recent past. These data sets are retrospective; relying on them to make forward-looking estimates does not capture the recent changes in customer awareness/preferences, model availability, and battery technology. Utilities, ISOs (Independent Systems Operators), and planners responsible for meeting and managing future EV charging demand are best served by a forward-looking approach that captures customer preferences and peer effects.
Do any of these methods account for human behavior and influence? For example, if a family member, friend, or colleague buys an EV and gives me a ride or even lets me drive it, I gain a greater understanding of EVs directly or vicariously – which could have an influence. As EVs become more common, more of this type of influence could affect purchasing habits.
Only AMB captures these effects – which are termed “peer effects” – probabilistically and from the bottom up. ABM models the discrete preferences, interactions, and evolutions of millions of individual agents (simulated people!) over time and excels at capturing that human behavior and influence piece of the puzzle. I mentioned this earlier but it’s worth emphasizing again. Research has shown that “peer effects” play a significant role in EV adoption behavior within populations, noting that “exposure to EVs at home or at work creates a ‘peer effect’ that drives consumers to more readily buy electric vehicles.”
This makes sense when you think about it…if you’re not sure what the EV driving experience is like, or about how or where you’d charge it, but then your neighbor or family member gets an EV and you’re able to ask her some questions and get some firsthand information from a trusted source, odds are you will be more comfortable with the idea of an EV the next time you’re looking for a new car. This peer effect is additive, working in tandem with the customer preferences and other demographic drivers that also factor into the decision of whether to adopt an EV.
(Fun fact: The same peer effect has been observed for rooftop solar adoption, a topic I wrote about in Zondits back in 2016!)
Well, this is all very interesting. What are the next steps in the evolution of this type of forecasting?
That is a great question. We have a number of ideas about future directions.
- Over time, we plan to move toward even more realistic decision-making that builds on additional customer research and reflects the rapidly evolving utility EV program landscape.
- We’re also building out our load forecasting capabilities to align with other tools/platforms and offer clients a “one-stop shop” experience.
- Longer-term, we intend to extend this forecasting framework to other residential equipment, such as heat pumps. We believe our EVAF’s strengths – geographic granularity, simultaneously capturing customer preferences and demographic drivers, and modeling peer effects – will be a powerful combination for heat pumps and other technologies.
Walter, thanks again for talking with Zondits. Given the rapid pace of developments in the EV industry, it’s easy to recognize the value and need for accurate adoption forecasting.
The original content of this article can be found here: https://www.zondits.com/zondits-interview-ev-adoption-forecasting-hows-that-done/