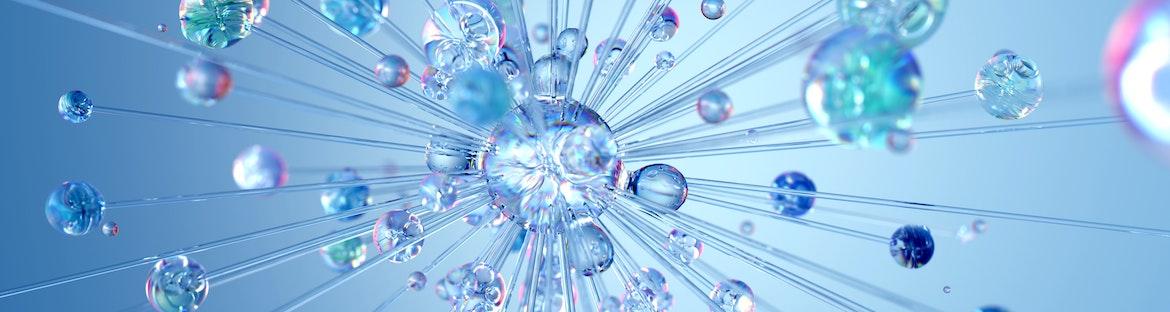
To scale AI beyond the pilot phase, organizations must anticipate and overcome the most common roadblocks and incorporate proven best practices into their strategies.
Driven by the falling costs and increased accessibility of AI platforms, besides the proven business benefits, more enterprises are integrating these technologies into their product innovation strategies. According to IBM’s Global AI Adoption Index 2022[1], 35 percent of companies are currently using AI—a four-point increase over 2021—and an additional 42 percent are exploring artificial intelligence solutions.
Once an enterprise has successfully implemented AI in one area, the next logical step is replicating the initial success in other areas. However, in a recent Forrester study commissioned by IBM, an astounding 90 percent of decision-makers admitted having difficulties in scaling the number of AI use cases across their organizations[2].
Why the speed bump between pilot and scaling, and what can businesses do about it? In this article, we’ll explore five of the most common roadblocks that can stall AI scaling strategies—and offer insights on how to overcome them—as well as some of the best practices that enterprises have leveraged in driving their initiatives forward.
Why scale AI now?
In today’s economy, many businesses are facing a slowdown in customer buying behavior, and adjusting to this reality often requires layoffs. As a result, previous product innovation strategies that would have been fueled by an army of personnel are now out of the question.
AI-driven technologies can enable teams across the organization to achieve more with the staff they have. By implementing an AI scaling strategy, enterprises can build on the momentum of their initial success, maximize the business value of their investments in the pilot, and leverage best practices while those “lessons learned” are still top of mind.
Scaling AI also uncovers opportunities to solidify and expand an organization’s competitive edge. According to Forrester, 50 percent of companies believe scaling AI will enable them to remain competitive[2], and 39 percent believe it will propel them to a leadership position in their industry.
What does AI at scale look like?
Most organizations make their first foray into AI to solve a specific business problem, such as long wait times in the call center. As organizational capabilities in AI mature, projects move on to encompass larger business challenges, like elevating the whole customer journey.
Gartner has created a maturity model[3] that neatly depicts the enterprise AI journey, beginning with Awareness (early interest in AI) and advancing to Transformational (AI becomes part of the business’ DNA). Each stage in this model represents a higher level of “AI at scale,” each delivering greater benefits than the one before:
Roadblocks to scaling enterprise AI
Once an enterprise has a proven success in enhancing products with AI, the obvious next step is to replicate the success elsewhere, and yet this is where many organizations stall out. Here are five of the most common areas where roadblocks can stymie efforts to scale AI in the enterprise:
Employee resistance
One of the biggest potential roadblocks in scaling AI is people-focused: many employees are apprehensive about adopting artificial intelligence because they fear their jobs are in danger. At this time, we can assert that AI has not yet evolved to a point where it can replace humans completely. To cite just one instance supporting this claim, as reported by Fast Company[4], CNET has been facing criticism since news came out that the tech outlet was publishing AI-generated articles under the byline “CNET Money Staff.” To make matters worse, Futurism found that not only did many of the articles contain errors, they sometimes plagiarized the work of human writers.
This is just one story highlighting the enduring need for “humans in the loop” when AI is implemented. Not only need employees not fear broad-based job cuts, but they also have the opportunity to broaden their resumes with skills such as managing AI bots, exploring new use cases, and implementing best practices.
The more effectively an enterprise can communicate to its employees how AI will—and will not—affect their jobs, the more quickly it can overcome this potential roadblock to successful AI scaling.
Data gaps
Enterprise AI systems rely on data, and if that data isn’t up to the task, efforts to innovate with artificial intelligence could hit a wall. At least half of businesses struggle with data-related roadblocks to AI scaling, according to the Forrester study[2]:
- 58 percent of organizations cite data quality as a major obstacle in scaling AI.
- 50 percent struggle with data integration for data science/machine learning and analytics/business intelligence platforms.
- 52 percent simply don’t know what their AI data needs are.
One error organizations make is thinking their data is ready for AI because they designed their data management capabilities to satisfy BI requirements. While there is overlap between the two areas, there are also significant differences. To ensure readiness for AI, not only must data be clean and current, but it also must be:
- Continuously sourced from enterprise applications.
- Governed and managed securely.
- Made accessible to AI teams.
Skill gaps
Alongside the right data, successful enterprise AI scaling requires the right people to implement, integrate, and manage the technology. Data engineering, data science, and developer skills are all in short supply; to fill the gap, firms are challenged to either upskill their existing personnel or hire from outside.
This is one area where establishing an AI center of excellence (see below) can ease the burden. By centralizing AI-focused talent in a hub designed to support all areas of the organization, businesses can make the most efficient use of the personnel they have—and free individual departments from the need to compete for their own talent.
Technology gaps
Of course, people need the right tools with which to work, and the gap between what enterprises have and what they need can be a hindrance to AI scaling. Fifty-eight percent of organizations say they lack the tools and platforms needed to build advanced analytics and machine learning models[2]; 50 percent lack the solutions to operationalize these models, and another 50 percent lack the solutions to monitor models in production.
Fortunately, firms that have already executed a successful AI implementation will have at least some tools necessary to scale. When deciding which area to focus on next, enterprises may want to prioritize those that can easily leverage existing technologies.
Cost- and time-related challenges
As successful as an enterprise’s pilot program may have been, some outcomes may cause hesitation in implementing AI elsewhere. In the Forrester study, 56 percent of respondents noted that the project cost of their initial AI implementation was higher than expected[2], and 41 percent affirmed that the project was delivered late.
Instead of allowing time and budget overruns to become a hindrance to scaling, businesses can chalk them up to “lessons learned” and incorporate this knowledge into their project plans for the next enterprise AI implementation.
So, how do you scale AI in an organization? 3 best practices
When we work with clients on scaling AI in their organizations, we implement proven best practices that can advance progress towards their goals while making the most efficient use of their resources.
1. Choose the optimal area(s) for expansion
When enterprises look at scaling AI, the obvious first question is “where next?” To maximize the chances of success, we look for areas where AI would be:
- Easy to implement.
- Likely to be accepted by employees.
- Has enough data available for model training.
- Has the potential to deliver solid business value.
2. Create a campaign for building momentum
With an AI scaling plan in place, the next step is to “take the show on the road” and start building support across the organization. Build a pitch deck documenting the successes of the pilot project, with an emphasis on how AI is making team members’ lives easier (for employees) and/or how it’s delivering business value (for leadership). Then meet with key stakeholders at all levels, beginning with leadership, and build a team of evangelizers who will help carry the AI message.
This support will be critical not only in procuring the necessary funding for the next enterprise AI implementation, but for initiating a cultural shift in which employees embrace AI as a net good that can improve the employee experience, create happier customers, and deliver solid benefits to the bottom line.
3. Build AI into the organization
Even in the early stages of AI scaling, firms can put into place the structures that will eventually support multiple AI iterations in multiple areas of the organization.
An AI center of excellence (CoE) enables businesses to consolidate AI-specific talent and resources in a hub capable of providing governance, advisement, and project oversight across the organization. A well-managed CoE can help the organization stay on course in pursuing its AI scaling goals by:
- Standardizing processes based on lessons learned in the pilot phase.
- Suggesting and prioritizing new areas for AI implementation.
- Prescribing the people, processes, and technologies necessary to launch AI projects.
- Creating roadmaps for AI implementations.
The end goal: becoming an AI-driven company
While artificial intelligence and machine learning are certainly nothing new, their growing prevalence across business processes is complicating the mission to protect personal data. By following proven best practices—and keeping an open ear for future strategies—organizations can continue reaping the benefits of AI-driven applications while preserving the trust of their customers as well as their compliance status with respect to data privacy laws.
About the author:
Paul Lee is a Senior Manager in Logic20/20’s Digital Transformation Consulting practice, with experience in digital, customer experience strategy, and technology implementation engagements.
Logic20/20 is a Solution Provider Member of Utility Analytics Institute.