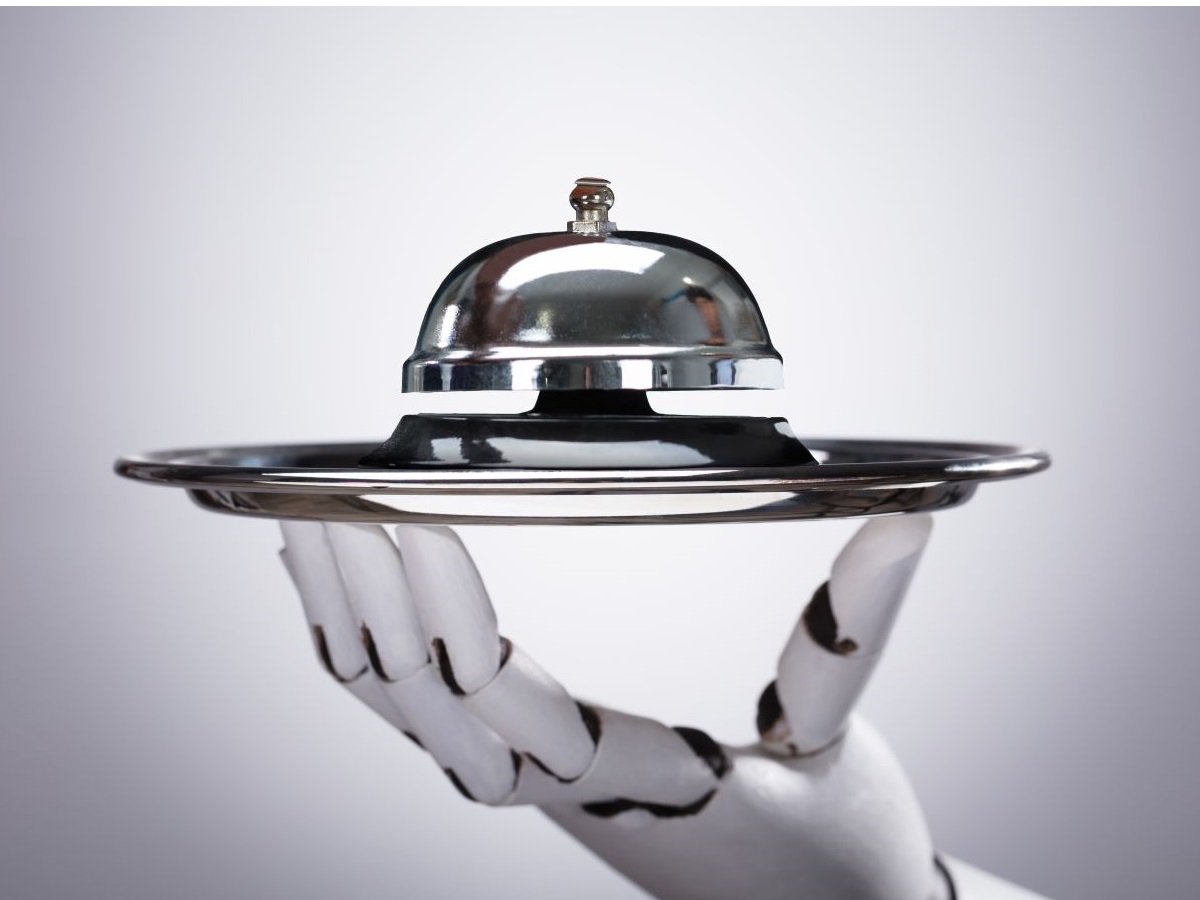
What is Generative AI? And how can organizations define value to focus their development efforts?
Generative AI has captured the imagination of businesses worldwide. It’s been hailed as a game-changer, a technological marvel capable of transforming industries. Yet, amid the excitement, the true nature and practical applications of Generative AI can sometimes get lost in the hype. This article aims to clarify Generative AI, define its strengths, and demystify its limitations. By understanding what Generative AI entails, organizations can harness its power to drive innovation and growth.
Generative AI
At the heart of the Generative AI buzz lies a fundamental question: What is Generative AI, and what do people mean when they refer to it?
Fundamentally, Generative AI is a diverse set of algorithms leveraging various underlying techniques to generate data or content autonomously. Generative AI foundational models are trained on massive datasets, contextualizing the underlying patterns and empowering them to create new text, images, audio, and even video.
However, the Generative AI buzz often centers around large language models (LLMs) and web-based applications. While indeed a remarkable feat of technology, these tools are just one facet of Generative AI.
LLMs are highly proficient in text-related applications such as translation, information retrieval, summarization, generation, and search. These capabilities make LLMs valuable in content creation, chatbots, and different business applications.
However, they fall short in other areas, like precise mathematical calculations or understanding real-world context. It’s important to dispel the misconception that Generative AI can work miracles in all scenarios.
Leveraging LLMs for text-based use cases
One of the most tangible applications of Generative AI lies in its ability to generate human-like text. Businesses can harness this capability for creative content generation, enhancing customer engagement in virtual agent interactions, and even automating some aspects of customer communications. For instance, chatbots powered by LLMs can provide real-time support, answering customer queries efficiently.
Other popular examples where Generative AI has shown tremendous potential are summarization and providing a natural language interface to perform data analysis and deliver value in efficiency across organizations.
In content generation, LLMs can create articles, reports, and marketing materials, saving time and resources. Human editors can then fine-tune the generated content to ensure alignment with the brand’s voice and messaging.
The limits of LLMs
While LLMs shine in text-based scenarios, they have limitations that must be acknowledged. They are generally unsuited for tasks requiring precise calculations or a deep understanding of real-world context. For instance, using an LLM to predict the exact impact of a distributed energy resources on system reliability is likely to yield unreliable results.
Understanding these limitations is crucial for organizations considering Generative AI adoption. Businesses should consider using this technology where it excels and seek alternative solutions for tasks outside its scope.
There are also risks worth consideration, which include but are not limited to hallucinations, intellectual property laws, and misalignment from core values.
LLMs providing access to data insights
One of the hidden strengths of LLMs is their ability to serve as gateways to text-based data. Businesses can extract knowledge from their data repositories more effectively by posing questions in natural language, reducing the need for advanced analytics skillsets.
This access to data-driven insights can empower organizations to make informed decisions, identify trends, and respond swiftly to changing market dynamics. It bridges the gap between AI and actionable business insights.
However, this will typically require some form of analytics on the underlying data before it is exposed to the model. Predictive Maintenance is a simple example of this architecture.
Debunking the myth of Generative AI for predictive maintenance
A common misconception is that LLMs will provide an end-to-end solution for predictive maintenance. While using AI for predictive maintenance is sound, expecting LLMs to perform tasks like predicting when a piece of machinery will fail is unrealistic.
Predictive maintenance relies on machine learning models trained on instrument-specific sensor data or historical performance metrics. When properly developed, these models can accurately forecast maintenance needs, optimizing operations and reducing downtime.
Once this analytics step is accomplished, LLMs can be applied to the outputs to allow less technical professionals to make natural language inquiries about the status of the machines.
Incorporating LLMs can enable access to a broader user group, but they do not solve the underlying analytics requirement.
Building trustworthy AI
In the world of Generative AI, building trust is paramount. Ethical considerations and transparency are central to the responsible development and deployment of AI technologies. Organizations must adhere to trustworthy AI frameworks and guidelines to ensure that AI applications serve their intended purposes while minimizing risks.
Generative AI – A path forward
For organizations venturing into Generative AI, maintaining a comprehensive strategy is essential. The strategy should be well documented and include information on AI system components, compliance structures/procedures, and guidance on best practices in AI development and deployment.
Navigating the evolving landscape of AI regulations is dynamic, but a well-maintained dossier can provide the necessary documentation and demonstrate commitment to responsible AI usage.
In conclusion, Generative AI, particularly LLMs, presents exciting organizational opportunities. By understanding its capabilities and limitations, businesses can harness its true potential and ride the technology wave into the future.
Visit the Deloitte AI Institute to learn more.
Deloitte is a sponsor of Utility Analytics Week 2023, brought to you by Utility Analytics Institute and produced by Endeavor Business Media.
About the Authors
Eddie Newland is a specialist leader at Deloitte Consulting LLP.
Subhani Syed is a senior manager at Deloitte Consulting LLP.
About Deloitte
Deloitte refers to one or more of Deloitte Touche Tohmatsu Limited, a UK private company limited by guarantee, and its network of member firms, each of which is a legally separate and independent entity. Please see www.deloitte.com/about for a detailed description of the legal structure of Deloitte Touche Tohmatsu Limited and its member firms. Please see www.deloitte.com/us/about for a detailed description of the legal structure of Deloitte LLP and its subsidiaries. Certain services may not be available to attest clients under the rules and regulations of public accounting.
This publication contains general information only and Deloitte is not, by means of this publication, rendering accounting, business, financial, investment, legal, tax, or other professional advice or services. This publication is not a substitute for such professional advice or services, nor should it be used as a basis for any decision or action that may affect your business. Before making any decision or taking any action that may affect your business, you should consult a qualified professional advisor. Deloitte shall not be responsible for any loss sustained by any person who relies on this publication.
Copyright © 2023 Deloitte Development LLC. All rights reserved.