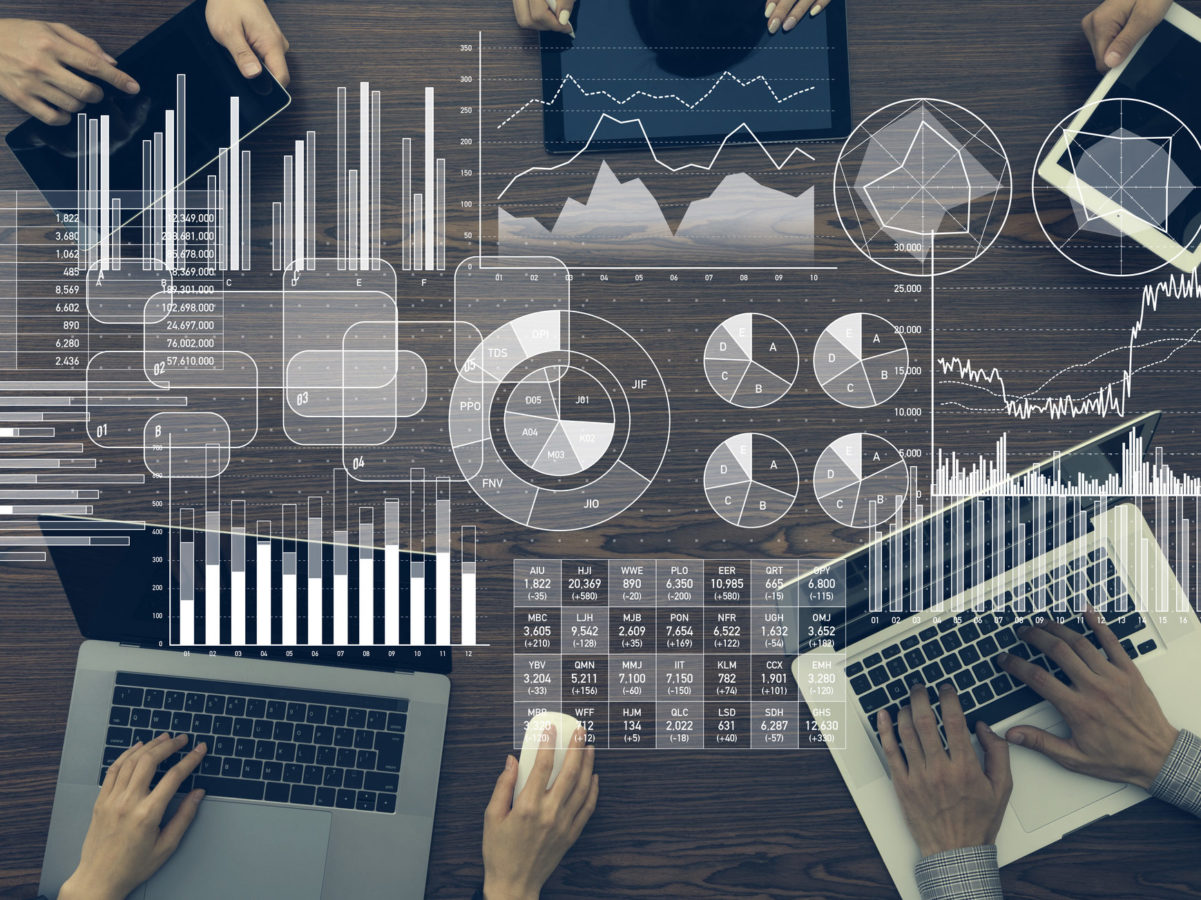
UAI’s Analytics Architecture & Technologies group is exploring strategies for publishing analytics models. R Shiny has recently risen to the top at many organizations.
Data visualization tools have brought many benefits to the utility industry, making it easier for employees to understand historical trends and events as they unfold. Advanced analytical tools enabling predictive analytics, optimization modeling and risk simulation help utilities predict what may happen, understand the impacts of possible scenarios, and assess what approaches to take.
However, there is still a missing piece. According to an expert from one utility IT business unit, “The last mile – how we help end users visualize the analytics model in context – has always taken a back seat.”
Anyone who has ever spent time analyzing data and delivering the results has likely had the following experience: the recipient asks, “How does the model work? What happens if you change this assumption or those inputs? Can we run the model ourselves?” The reply of the analyst is usually, “Do you have analytical applications? Can you code?”, and then, crickets.
There is a whole new generation of analytical tools, such as opensource R and Python, and advancements in more traditional tools like SAS and SPSS that make modeling easier. Even better, there are tools that give the internal customer (the business) a way to “play” with models by manipulating various factors and inputs.
In the UAI Analytics Architecture and Technology Advancement Group[1], the advanced analytics team at one southeastern utility has shown just how useful these tools can be. This group created a self-service infrastructure in house, which allows analysts in their group and throughout the business to create, publish and maintain web applications. Shiny, a product in the R Studio, is one of the tools being deployed. The analytics group “owns” the management and maintenance of the web applications. IT ensures the security of the model outputs – only approved personnel get access – and provides data governance.
The advanced analytics group wanted to be in sync with the company’s strategic analytics goals, particularly to establish common platforms. According to the manager of the group, “It is bit risky to try to pick one platform or tool and say this is what we are going with, especially in an area like analytics where things are moving so quickly. At the same time, we didn’t a want situation where every group is using their own unique tools and platforms for storing, analyzing and managing data and results in ways that aren’t accessible to others.”
The list of use cases that the group has enabled is impressive. Web applications are being used to evaluate marginal impacts of generation assets, predict employee retirements, and simulate the probability of meeting debt targets.
The group tracks page views by application and over time to understand which applications are the most trafficked. By far the most frequently used application is the assessment of peak load risk. The application goes beyond traditional deterministic load forecasting. It enables the users to estimate uncertainty bounds and probabilities (loss of load, challenging reserves) using regression and Monte Carlo simulation.
The Shiny deployment meets a need that enterprise analytics platforms don’t – a way to bring advanced analytics to the thousands of meaty questions that arise in running a utility business.
Of course, there are limitations. The deployment is not meant to operate at the scale and robustness of an enterprise analytics platform. Nor is it the best tool for data visualization (most competitive data visualization tools are much quicker to set up and have better graphics).
Regardless, the benefits stand out. According to a senior IT manager at the utility, the Shiny deployment has shown benefits. “The models developed so far are unique and useful to a group of people that ask for it. These kind of use cases will continue to increase. This deployment has an important place in the whole data analytics strategy.”
[1] All members of UAI Advancement Groups will be referenced anonymously to protect their privacy and security.