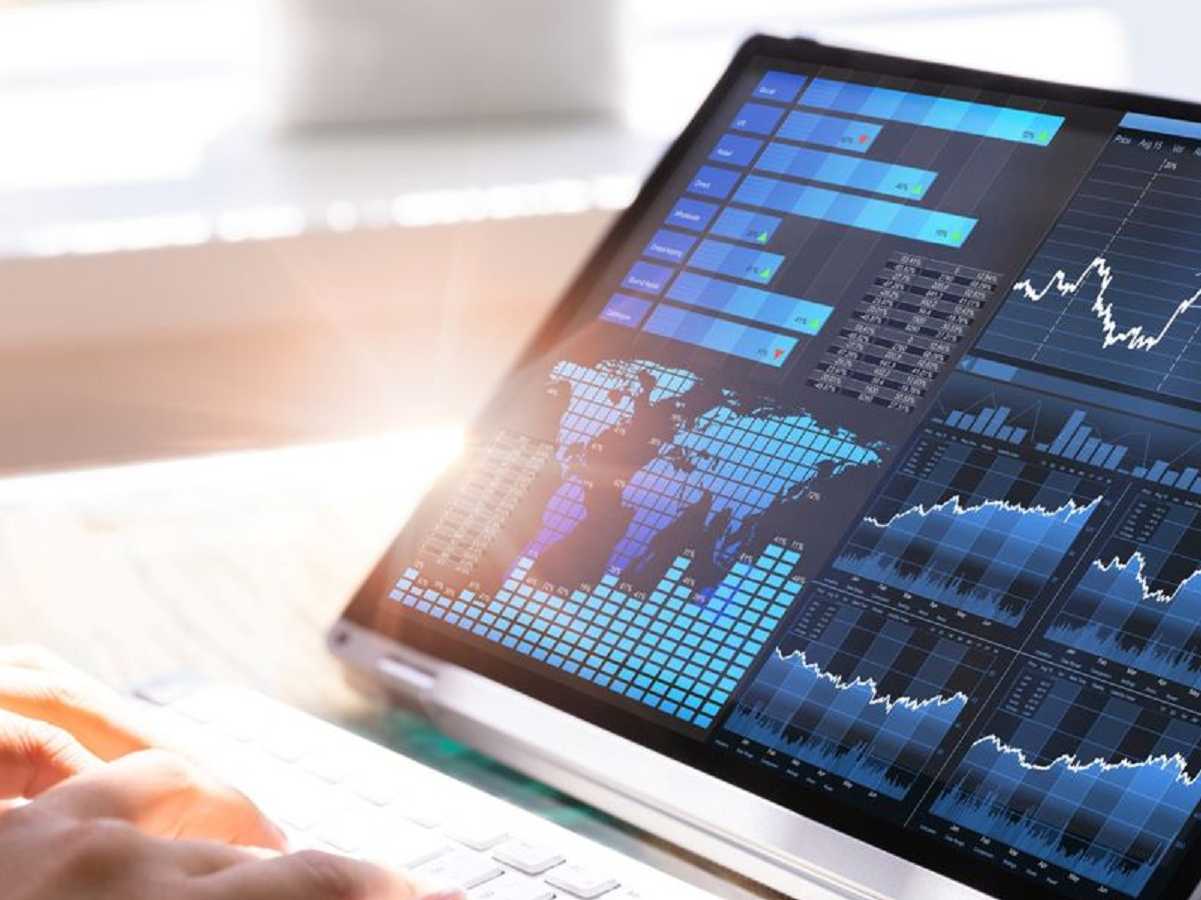
Predictive analytics can help utilities realize value through improved reliability, decreased O&M costs, improved safety, and developing a coherent, systematic approach to asset base replenishment.
Using analytics for asset management within utility operations is far from a new concept. The power delivery system represents one of the most asset-intensive industries, and utility leaders have been seeking ways to leverage available data to achieve improved reliability and reduce O&M costs. Many asset managers are challenged to put into practice the necessary processes and technology, often relying on spreadsheets and legacy software that was never intended to provide actionable insights. The proliferation of new technology, sensors, and data historians has generated more data with potential to improve asset management.
The utility is often challenged with asset issues such as:
- How do we allocate our limited budget to focus on the highest risk assets (e.g., failure risk or safety risk)?
- Which assets are at most risk of failure that could be most easily predicted?
- Do we replace earlier prior to failure or wait until failure to replace?
Predictive analytics for utilities
Predictive analytics for asset management seeks to leverage available data sets to optimize the utilization of assets to maximize useful life and value to the operation. When implemented properly, predictive analytics can help utilities realize value through improved reliability, decreased O&M costs, improved safety, and developing a coherent, systematic approach to asset base replenishment.
Moving from a run-to-failure approach with assets to a condition-based approach which can leverage predictive analytics, can transform utility spending by reducing O&M costs and providing opportunity for more strategic capital spending. (Figure 1)
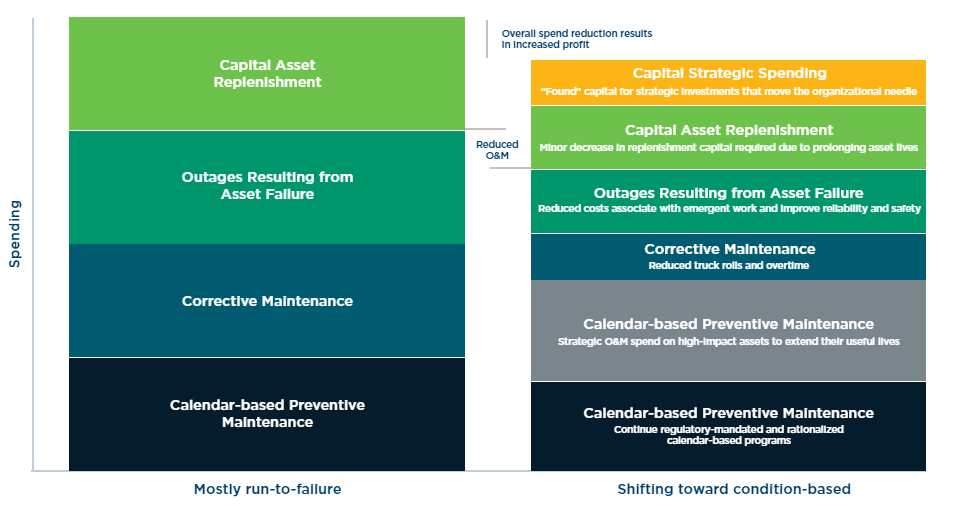
Figure 1. Overall Spending Transformation
As utilities seek to leverage predictive analytics for asset management, fundamental processes and field practices are needed to achieve successful outcomes. By putting better processes and practices into place today, asset data can be better organized and more accessible to apply analytics in a way that is actionable, measurable, and delivers a return on investment (ROI). If a utility deploys such improvements, they can see millions in savings – Duke Energy avoided >$130M in costs thanks to OSIsoft monitoring systems and condition-based maintenance practices.
Common obstacles to implementing predictive analytics for utilities
Figuring out where to begin with implementing predictive asset analytics can be a daunting task:
- Which assets should I use analytics on?
- Is there enough data on my assets to support analytics?
- What data could be acquired that would enhance prediction?
- What new tools or processes could be put into place to enable better data collection on asset failure to inform asset analytics efforts?
- Which departments need to be involved in the initiative and how is alignment/buy-in achieved?
Considering the influx of data on each asset, if the data is unstructured, inaccessible, or not relational, it can be overwhelming and difficult to use for analytics. For example, failure data related to outage events is often sectionalized by protective section, not just at the feeder level. Additionally, some data on a particular asset might be kept in an Enterprise Asset management System (EAM) whereas other data is maintained in GIS and work requests related to that asset are in a third system.
It is important then to build the enabling foundation for analytics by improving processes and tools which can improve existing analytics and prepare for future analytics initiatives. Some examples of how process or tool improvements can enable analytics resulting in benefits to the utility or customer are shown below.
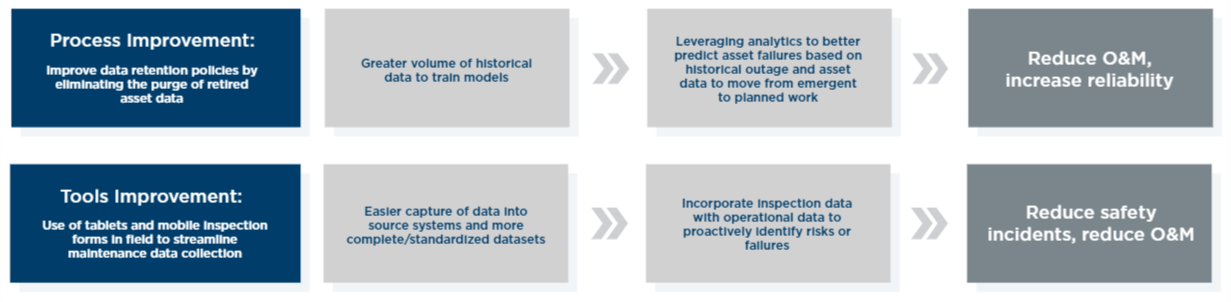
Figure 2. Examples of value from process or tool improvements
Building the foundation
The proliferation of data is often viewed as one of the greatest opportunities for utilities, but some might say that opportunity also presents one of the greatest challenges– harnessing data to create value (e.g., greater return on capital, fewer/shorter outages, fewer safety incidents). The foundation for asset analytics starts with the strategy. The asset analytics strategy should address both the use case prioritization – including the business case for and road map to achieve a use case – and the processes and tools needed to enable analytics value across use cases.
Not all utility assets are created equal or warrant the same amount of attention. A one-size-fits-all approach to utility asset management is not feasible. Across different asset classes, variations in maintenance requirements, monitoring capabilities, failure modes and regulatory directives may drive differences in strategy.
Also, within asset classes, the geography, grid location, environmental conditions, vendor, age, material, and other factors will drive differences in probability of failure and impact of failure. Therefore, utilities should tailor approaches and prioritize asset classes where the most business value can be realized.
Determining which assets to focus on is typically a function of the utility’s ability to collect consistent and relevant asset information while also considering the criticality of the asset to maintain vital utility operations. Despite relatively high criticality and benefits of using predictive methods, the level of effort to implement (including data collection, integration, and analysis) can sometimes outweigh the benefits.
For example, distribution transformers, substation assets, and distribution automation assets are typically considered to have a high potential for predictive analytics due to the ability to leverage real-time information and data feeds from AMI/SCADA. Additional predictive analytics can be explored for other assets such as poles, conductors, non-distribution automation switchgear and others, but they typically require more effort collect and manage data.
After the value from analytics for an asset class is identified, the utility can define what characterizes good and poor health for that asset. Then, as described in the distribution transformer example above, the utility can work with SMEs to inventory the available data associated with that asset class – e.g., EAM data, real-time data, maintenance inspection reports – that can be leveraged to build a failure model for that asset. This inventory of data can help to estimate the level of effort to implement a specific use case.
Business case analysis is an effective way to align analytics use case efforts and/or determine whether it makes sense to build or buy a solution. But the journey doesn’t end there. Look for Part 2 in the following issue, where we’ll discuss process and tool challenges, data acquisition, data governance, and organizational alignment.
This is the first part of a two-part series. Read part two here.
Authors
Eric Anderson, Senior Manager, West Monroe
Kevin Hade, Manager, West Monroe
Kojo Sefah, Senior Consultant, West Monroe