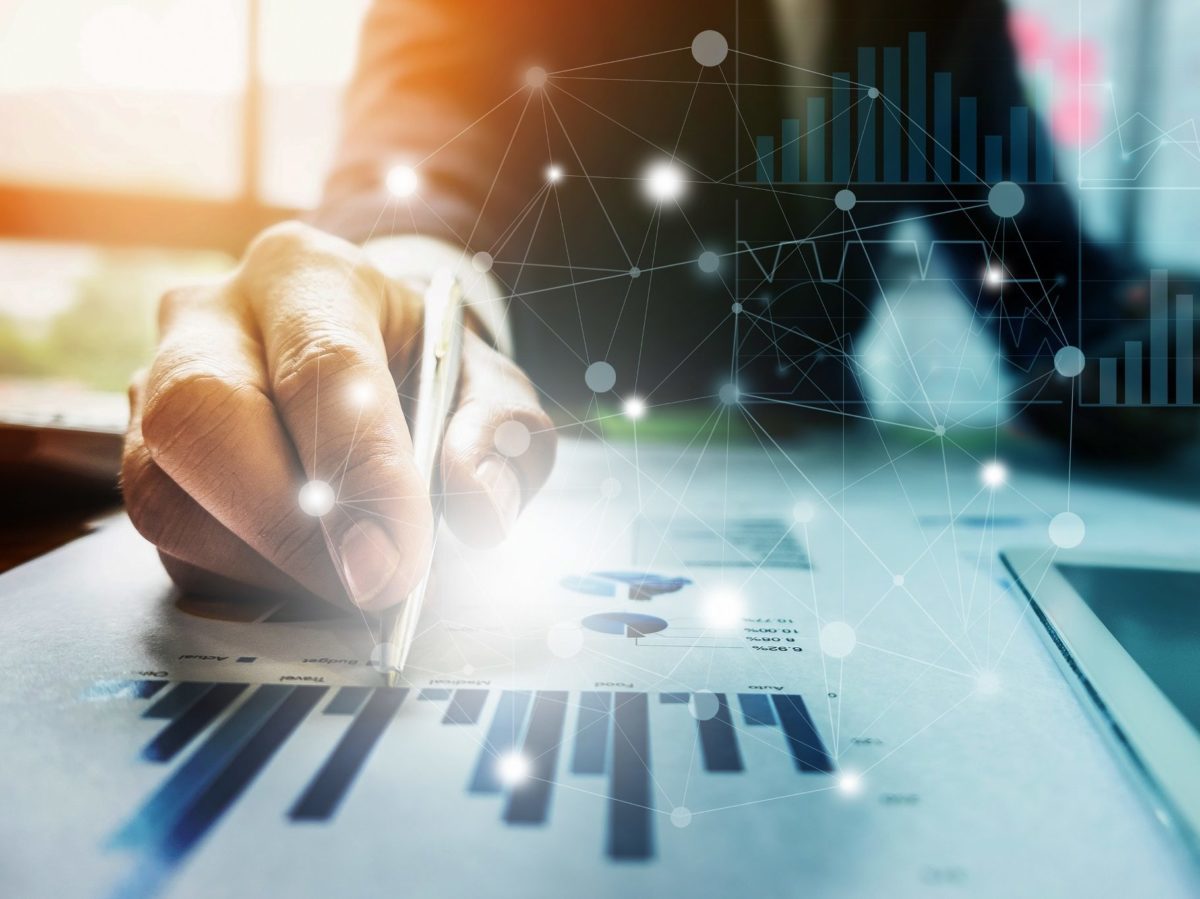
Data governance is like motherhood and apple pie. Everyone thinks it is a good and necessary thing. Should the pervasive use of analytics change how data governance is done?
Stakeholders at leading utilities interviewed for Ensuring Success in Analytics: A Playbook for Utility Executives, reported that data governance is not optional when it comes to analytics. What’s changed since then? It’s difficult to conclude whether utilities have made progress on data governance since that report. With utilities, as with other industries, few companies collect and report metrics on the progress of data governance initiatives.
A little background on data governance. Broadly speaking, data governance is the management of data throughout its life cycle. Data governance includes the people, processes and technologies needed to guarantee generally understandable, correct, complete, trustworthy, secure and discoverable data. There are a lot of categories under the data governance umbrella (See figure 1).
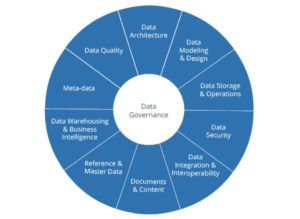
Source: The DAMA-DMBOK2 Guide Knowledge Area Wheel (source: https://www.dama.org/sites/default/files/download/DAMA-DMBOK2-Framework-V2-20140317-FINAL.pdf)
Since 2018, there have been few, if any, studies on the progress with data governance at utilities. However, here’s what utilities are saying these days about data governance and analytics.
Data security and privacy must be baked into self-service.
More and more, utilities are striving for a data-driven corporate culture. Think PPL, Exelon, Duke, and Consumers. The push is on for self-service. The idea is to offer data up beyond Agile analytics teams that typically have access privileges to others in the organization that don’t.
Privacy becomes an issue when personal identifying information (PII) is involved. Having policies on security and privacy as a part of data governance is one thing. Having them work on a day to day basis is another. Data governance privacy standards need to be operationalized. One way to do that is to embed governance into tools used for self-service analytics, such as data catalogs, so that private information is not exposed.
Analytics initiative success depends on the credibility of data.
Data quality is on the top of the to do list for data governance related to analytics. On the way to a establishing a data-driven organization, there will skeptics. When suggested actions delivered up by analytics are not what they are used to doing, the first questions usually are “What data did you use? It is high quality?” To get buy-in for analytics results, data quality is important. [Note: Change management also helps].
Sometimes the application of analytics delivers results that appear to defy the laws of physics. Utilities run critical infrastructure, so, in these cases, skepticism is healthy. That’s why validation is a necessary step, not just at first the first use of a tool, but on an ongoing basis for subsequent experimentation. This is especially true when using relatively new analytics such as AI, governance should include validation and verification practices. Note the on-site investigations deployed at Duke to verify and validation the momentary outage findings from their experimentation with deep learning self-organizing maps (see What Deep Learning Can Teach Utilities).
There are many situations where the data quality is poor. According to one former utility analytics executive, “The organizational commitment to data governance at utilities has gotten better at insuring that data is being populated correctly when setting up an asset in the system. For example, when a transformer is originally installed, we have the serial numbers, capacities, locations, etc. But when a storm comes through and a transformer needs to be replaced quickly, we aren’t as good in capturing replacement components. Analytics may show that the transformer is overloaded, when it might have been replaced with a transformer with more capacity.” That is where data governance needs to clearly prescribe methods for insuring quality through the data lifecycle.
Business helps define the right data to share.
It is also important that data governance be embedded into the utility analytics culture. It is not just an IT exercise but requires business involvement in data stewardship. There needs to be a feedback loop between analytics teams and those responsible for data governance. Efforts that start with the definition of a critical use case as well as identify and classify the right data to analyze, will help promote efficient data sharing within the organization. And after all, that is the point of data governance.